Research Leaders' Playbook
Tools and tactics for leading coordinated research programs
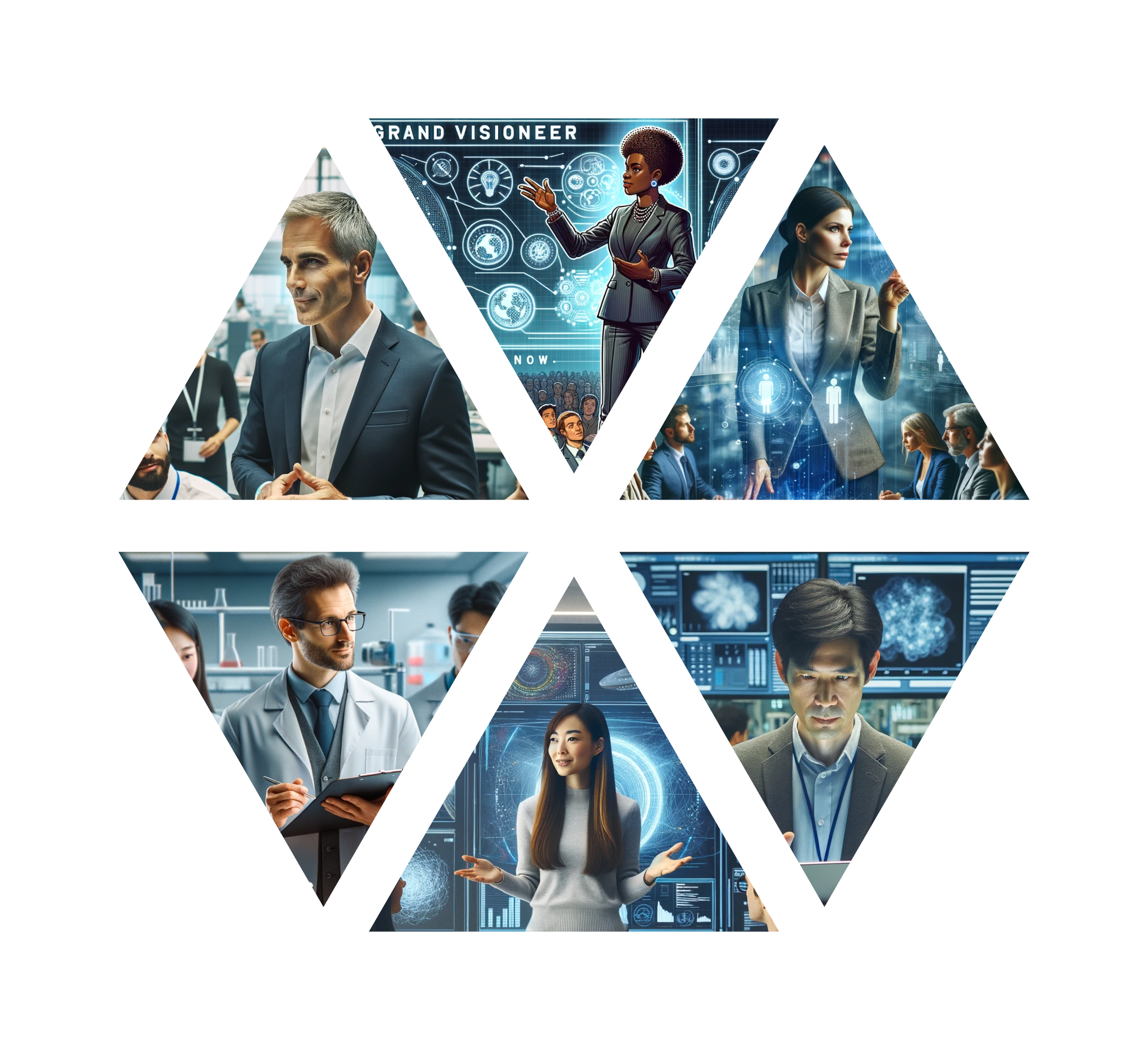
Table of Contents
-
The Different Roles and Phases of Running a Coordinated Research Program
-
Institutional Moves for Interfacing with Other Organizations
The Different Roles and Phases of Running a Coordinated Research Program
The leader of a coordinated research program[1] needs to wear many hats. This is one of the reasons why the job is so hard! These hats roughly correspond to the different phases of a program. Leading a program through each phase is almost a different job.
It's important to note that these phases are not rigidly sequential. They bleed into each other and you need to work on several at the same time: it's important to consider tech transition even while doing exploration and field mapping --- keeping your eyes out for who will shepherd the technology beyond your program and what those people care about; you will need to update both your program design in real time as you execute on it and run into previously unknown unknowns; you should have a communicable vision from day one and update it as you explore and refine ideas.
Some write-ups that don't fit into any specific role or phase
1. Exploration and Field Mapping
The goals of exploration and field mapping are to:
-
Have a working knowledge and network of the people and organizations working adjacent to your program's goal
-
Understand current practice well enough that you can evaluate people and ideas: who is good; who is moving slowly because they are slacking and who is merely working on something incredibly hard; whether you are asking performers to do something impossible or merely very hard.
-
Understand the limitations of current practice well enough that you can go several layers deep and identify unintuitive gaps or bottlenecks (see Identifying bottlenecks and Key risks).
Your role here is similar to a startup founder doing customer exploration.
You probably come into a program with a hunch. If you don't; create one. Exploration without an initial hunch to at least bias your search is far less effective. The point of a coordinated research program is to reach a goal. It's tempting (and a commendable urge) to leave yourself completely open to serendipitous goals. It's counterintuitive but having a goal makes it more likely to find different goals because you have something to compare to and contrast against.
Field mapping is the process of figuring out the state of a "field" --- the rough interconnected ideas. Who is working on what? What things are not being worked on? Why?
Some concrete tactics for exploration and field mapping:
-
Creating a list of research programs that have attempted something similar in the past and talking to the people who ran those projects. (If you think that nothing like what you're thinking about doing has been done, you're wrong.)
-
Creating a list of 100 researchers and companies that seem to be working in or peripheral to your goal and talk to them.
-
Reading a few review papers in order to identify people who might be good initial nodes in a network to reach out to is a reasonable starting point,
-
Writing down intermediate syntheses, hypotheses and conclusions from conversations in long-form text. Long-form writing (as opposed to bullet points) is important because it enables you to create causal connections between different things that you're exploring and to notice unintuitive gaps or consistent blockers, both of which can be fruitful things for a program to go after. Update these notes as you learn more. Linked notes tools like Bear or Obsidian can be useful for this, but Google Docs, paper, or the built-in notes tools in Windows or Mac are fine --- whatever works best for your brain.
All of these require navigating a network
It's tempting to do an exhaustive search of all potential people and ideas in a space, but this is a bad idea. This phase of the program should be as short as possible, but no shorter! A good heuristic for when you've done enough exploration and field mapping is when your network exploration starts to close loops. That is, you start being routed towards people/papers/organizations by conversations in seemingly very disparate areas.
Related write-ups
2. Refining Ideas
The goal of refining ideas is to identify:
-
A concrete program goal that is both incredibly aggressive and potentially achievable within the program's lifetime.
-
Milestones that would suggest the program is moving towards that goal.
-
The different thrusts that a program would need to address that goal.
-
The key risks to the program (see Identifying bottlenecks and Key risks) and what evidence would suggest that you have mitigated them.
Refining ideas requires both thinking and talking. You need deep thinking to connect and distill the pieces of information that you've gathered while exploring and field mapping, and then you need to talk to people to test those ideas against the world.
Your role here is an analyst and researcher.
Refining ideas should happen in a loop with exploration: some conversations generate an idea for an impactful sub-goal and then running that idea by those same experts (or different ones) can give you more unintuitive nuance on it. Remember, people default to talking about higher levels of abstraction so it's up to you to make the ideas more precise.
Some concrete tactics for refining ideas:
-
Running workshops
-
Requests for information
-
Circling back with people you talked to during exploration with a concrete hypothesis for them to react to
Related write-ups
3. Program Design
The goal of program design is to create the structure of a program:
-
What work actually needs to be done to achieve the program's goals?
-
What kind of projects are best suited to encourage that work?
-
How do those projects need to interact?
-
What needs to happen at the end of a program so that it can have impact? Working backwards, what do you need to put in place during the course of the program so that can happen?
Your role here is a strategist, laying out the troop movements.
Some examples:
-
You might determine that your program's goal can be achieved with widely-available resources combined with a hard-to-create dataset. In this situation, a good program design might consist of one or more projects to create the dataset combined with a competition. (This is roughly the shape of the Scroll Prize<link>) Those projects and the competition need their own design (how do you know they're successful, what timelines do they happen on, how will the competition be run and managed?)
-
You might determine that there are two very different approaches that could potentially achieve the program's goal. One is higher at a higher Technology Readiness Level (TRL) but faces a lot of scaling risks, while the other is lower TRL but will clearly scale if it addresses early risks. In this situation a good program design might consist of one track that funds startups working on the high TRL approach and another track funding labs working on components of the lower TRL approach. The existence of the program will make sure that the labs coordinate towards a coherent output and that if the startups fail to scale the higher TRL approach, they might be able to adopt the other approach which the labs have matured.
Concrete tactics for program design:
-
Writing down the ideal end state for the program and a causal narrative in as much detail as possible for how that would feasibly happen.
-
Creating Block Diagrams.
-
Putting out requests for proposals.
Related write-ups and resources
4. Communicating Vision
The only way your program will be successful is if you are able to convince and align several different groups:
-
Great collaborators (performers, employees, etc.)
-
The people holding the purse strings
-
Whoever is going to carry the technology on beyond your program
Your role here is to be a keynote speaker, a general rallying your troops.
In order to get any groups on board with the program's vision, you need to have a clear idea of what it is. (This goes back to creating concrete goals).
Communicating the program's vision to collaborators is critical because
-
People who feel like they're working towards a shared vision do better work.
-
In part your program will succeed because people will continue to work towards its visions even after the program ends.
-
Even collaborators who are paying lip service to a vision because you can throw around serious chunks of money need to understand it to be effective. There are so many small decisions that can't be captured by metrics that they will fail to do good work (and you might have to pull their funding) if they don't know where that work is targeted.
Concrete tactics for communicating vision:
-
Always have an up-to-date...
-
One or two sentence blurb that you (or people who are making <double-opt-in intros>) can use in emails.
-
A one-to-two-page document that explains the current working ideas of your program.
-
A deck of slides that you can go to in order to illustrate specific ideas in your program that are easier to communicate visually.
-
-
After every conversation you have, write down:
-
Questions or points of confusion that came up
-
Which specific things you said that resonated or fell flat
-
Points of regret or things you could have explained better
-
-
Pay attention to the Pyramid Principle --- start with the bottom line of the program up front "we're working on tools to 10x the production of X" and then go into details.
-
Simplify your language as much as possible, but no further. Even technical people secretly like straightforward explanations and you will need to communicate across a number of disciplines. AI tools like LLMs can help with this.
-
Start with concrete examples and stories and then work your way to abstractions.
Related Sections
5. Program Engineering
Once you have a precise program design and your program is funded, you need to get into the weeds of who is doing what. This means soliciting proposals, negotiating contracts, and generally putting rubber to the road.
Your role here is a tactician, a salesperson, and a contract lawyer.
Ultimately, no plan survives first contact with reality intact. You will have to constantly adjust your program design based on who you can get on board and what their demands are once you start getting into specifics.
In this phase, it really pays to understand the nitty-gritties of the processes in your specific organization: what kind of contracts you can write, what the process looks like, and who is in charge of it. Doing this will allow you to have as much freedom and power as you can without getting sued, fired, or going to prison.
It's hard to give generic, concrete advice at this stage, but some meta-concrete advice:
-
Do the legwork to understand the specific constraints in your organization on what sort of contracts you can write.
-
Always keep planning for transition in mind, especially around IP.
-
Make friends with your legal team!
-
Read the fine print.
-
Everything is negotiable. Many organizations may say "this is how we do things. Full stop" but given the right incentives, that is never the case.
-
Negotiating contracts always takes longer than you expect.
-
Keep in mind that nothing is a done deal until the legal contract is signed and the money is out the door. (And even then, things can go south.)
The classic book out there on negotiation is Never Split the Difference, which is well worth reading before you get to this phase of your program.
Related Sections
6. Program Execution
Program execution is when the "actual" research happens: contracts are signed, money is out the door, and researchers are measuring, mixing, tinkering. Ironically, as the program leader there will not be as many things for you to do on a daily basis.
Your role here is as a project manager and CEO.
Treating projects within the program as "fire and forget" is often a mistake for several reasons.
-
Work expands to fill the time allotted to it. Without tight feedback loops with people doing the hands-on work, whether they are external collaborators or employees, things will often take longer in a compounding way. This isn't to say you should be breathing down their necks, but it's important to touch base regularly.
-
Success will often require making adjustments to the program over time. The more in the loop you are, the smaller those adjustments need to be to keep things from going off the rails. There is a tension here because you also don't want to micromanage.
-
Some of the power of a coordinated research program comes from cross-pollination between the different projects. It takes work to make sure that they are talking to each other regularly.
(Of course, the relative importance of these different factors depend on what sort of program you have and what kind of program leader you are.)
Some concrete tactics:
-
Have regular in-person check-ins with performers. It's one thing for people to say "everything is fine" on the phone but a very different thing when you can see the lab.
-
Have gatherings for any external collaborators with plenty of unstructured time.
-
Be willing to kill projects. It's incredibly hard, but can free up resources for other work.
-
Be willing to double down on projects that are going very well.
Related Sections
7. Tech Transition
In order for a program to have an impact, the technology needs to transition beyond the program. "Transition" can mean many things: continuing as an active area of research in other organizations; being incorporated into different products; spawning one or more startups; becoming an open source project; and many others.
Your role here is a business development person and advocate.
You need to start planning for this phase of the program long before the end of the program.
Realize that technology ultimately lives in people's heads --- the more people who worked on the technology as part of the program you can enable to continue working on it afterwards, the more likely it is to succeed.
Ultimately, you need to give up control over something that has been your baby for years.
You can see concrete tactics in Planning for transition.
Related Sections
The adventure begins
This playbook is a hopefully useful piece in a bigger toolkit that can enable you to lead amazing research programs. You can (and should!) complement this playbook with two other resources. First, do deep dives on the stories of previous programs -- both successes and failures -- that bear a family resemblance to what you're setting out to do. Second, find people who have done similar things before and talk to them about what you're trying to do, ideally more than once. Successfully leading a program requires many different hats at the same time. Some of these roles will inevitably be in tension with one another. The skill of being a program leader is knowing which tools and approaches to prioritize and when. Some of that can come from external sources, but at the end of the day, the responsibility of making that call rests on your shoulders. Good luck!
WTF is a Coordinated Research Program
"Coordinated research program" is, quite frankly, a made up term to cover a wide range of activities that nevertheless share enough important similarities that we can talk about them as a group. Like most nebulous things, any tight definition would have so many exceptions and caveats as to be useless. So instead, I'll define a coordinated research program by describing what it is and what it is not so you can start to pattern-match.
Coordinated research programs:
-
Do research work that doesn't make sense for a single academic lab or startup to tackle for some systemic, institutional reason. There are many reasons why it might not make sense: it might not fit in an academic lab because it requires a lot of repetitive engineering and wouldn't create publishable results; it might not fit in a startup because it would be hard to capture the value it creates or isn't creating a sellable product at all. Unfortunately "nobody will fund me to do this" is not a systemic, institutional reason.
-
Have a single, opinionated leader with a lot of control over the program's direction and actions.
-
Involve more than one person.
-
Are finite and have a precise goal. That is, when you get to the end of a finite amount of time, you know whether you've hit your goal or not. Coordinated research programs can certainly lead to other coordinated research programs in order to hit a longer-term goal, but each program needs to have it's own clear goals.
-
Very often create public goods. This is generally a big reason that they don't make a good startup. Some cases are obvious like creating an open-source tool or public dataset. However, there are many gray areas, such as a program that figures out how to scale up a process that a startup then commercializes. The general sense is that these programs should create some kind of positive externality, otherwise they either aren't worth it or should be funded by investors.
-
Very often involve work being done in more than one organization. Coordinated research programs exist on a spectrum between fully externalized, where the coordinating organization does none of the hands-on research, work to fully internalized where the coordinating organization does all of the hands-on research. In their platonic ideals, ARPA programs are an example of the former and FROs are an example of the latter.
Within these broad characteristics, this playbook is primarily meant for people creating coordinated research programs with a few more characteristics:
-
They are meant to have a broad impact in the world, whether it's through creating new knowledge, tools, or processes. Coordinated research programs can try to unlock esoteric new knowledge but the way you go about starting and running them is quite different.
-
Have a timeline of ~five years and a budget on the order of ~$10-50m. The way that one plans for drastically more or less time than five years or raises and deploys drastically more or less than ~$10m are just very different.
Some examples of coordinated research programs within the scope of this playbook:
-
ARPA programs.
-
Focused Research Organizations.
-
Many of the programs at foundations like The Gates Foundation or The Sloan Foundation.
Some examples of coordinated research programs at the edge of or beyond the scope of this playbook:
-
LIGO
-
The Rockefeller Foundation's Molecular Biology program
Some examples of things that probably aren't coordinated research programs:
-
Most NSF and NIH programs. Importantly, program officers are often beholden to committees about what they fund and have little ability to change the program's direction once it has started.
-
Most institutes (like the Alan Institute, the Arc Institute, HHMI etc). Importantly, institutes last an indefinite amount of time and often have broad rather than precise goals.
The Mindsets of a Coordinated Research Leader
There are many mindset shifts between most other jobs and leading a coordinated research program. It's impossible to enumerate all of them, but perhaps the top ten are:
-
Extreme urgency
-
Acting under uncertainty
-
Bias towards action
-
You are not a PI or a Startup CEO
-
Identifying what not how
-
Focusing on bottlenecks and key risks
-
Starting with the end in mind
-
Impact over novelty
-
Turning the impossible into the inevitable
-
If it doesn't 10x, it's not worth it
1. Extreme Urgency
Most coordinated research efforts, whether they are ARPA programs or FROs, only last around five years. That may seem like a long time, but if you work backwards from an ambitious goal (like showing that it's possible to make GPS units small enough to fit in someone's hand when they are currently the size of a shipping container), things need to start happening from day one.
Furthermore, you need to effectively transmit this urgency to those around you: it's not the normal mode of action for many disciplines or organizations and they will push back.
2. Acting under uncertainty
Acting quickly means making big decisions before you have complete information --- not only because you don't have the time to be 100% sure that an action is the best action, but because when you're doing ambitious work, it's often impossible to know what the optimal action is.
Concretely, this means not hesitating before you send that email over whether it's to the right person, or whether you've done enough background research.
3. Bias Towards Action
If there's a choice between an action and thinking more about a problem, go for the action.
Concretely, this means that the gap between saying you will do something and doing should be very small. Have that meeting or send that email on Friday afternoon instead of Monday; pick up the phone or visit a lab instead of playing scheduling ping pong; get the one project you're sure about started even if others are still up in the air.
4. You are not a PI or a startup CEO
The role of a coordinated research leader can look a lot like a PI of a lab or a CEO of a company but there are several key differences that you always need to keep in mind:
-
Performers and collaborators do not work for you. Almost all coordinated research programs involve working with researchers at other organizations, to varying degrees. With these people, you don't have the ability to specify exactly what gets done and the feedback loops around the work are looser than if everybody were in the same organization. External work will often be proposed to you, as opposed to you specifying exactly who should do what. Even if you have a specific contract with external collaborators, the only real lever you have is whether to continue or end the contract.
-
You rarely own the results of the program. The goal of a coordinated research program is to get the job done. If it were possible to do that while making money, you would have started a startup; if it were possible to do that as the first author on a paper, you could do it in an academic lab. A program might produce papers, IP, or companies, but if you focus on capturing those, you will hamstring the program's success. To a large extent, running a coordinated research program is a service position: your job is to enable others.
-
Your goal is not to build an empire. In most situations, your program and position will be temporary. You should not focus on building a legacy or a lasting entity -- the job is to reach a finite, concrete goal.
As such, you need to constantly be thinking how you can convince people that their goals align with your goals and create communities and systems that will continue beyond the short few yours that your coordinated research program exists.
5. Identifying What, Not How
In part because you are not going to be the expert in all aspects of your program and in part because research ideas come from unexpected places and evolve over time, your primary job is to identify what goals and pieces of work need to be done, not how to achieve them.
Concretely, that means identifying the key metrics that need to be hit, interfaces, and minimal system architectures but not becoming attached to any particular idea early in the program's lifetime.
The need to focus on what over how is especially important the more external researchers you are working with.
6. Focusing on bottlenecks and key risks
Thinking about how to enable technology that currently seems impossible can be overwhelming. One way to cut through the noise is to focus on two (connected) ideas:
-
Bottlenecks --- these are the problems/capabilities that, if solved/created, would enable many other people and organizations to move forward on the technology you care about.
-
Key Risks --- these are "sticking points" (especially facts about the world) that if true, would make your program's approach infeasible.
Creating projects to address bottlenecks and key risks is an effective way to move a program forward.
For more on bottlenecks and key risks, see this write up.
7. Starting with the end in mind
It is important to work backwards from not just your program's goals, but the broader technology impact you intend your program to unlock. These goals might mean a concrete technology or pathway to one, but it could also be a new understanding of what the actual problem is, so that a future program can solve it.
This working backwards needs to include not just technical steps, but human ones as well: what communities need to be formed, how will technology be adopted, etc.
8. Impact over novelty
It is tempting to focus on doing exciting new things that nobody has done before, but that isn't the role of a coordinated research leader. Yes, your program will need to do new things, but you should actually do the bare minimum of new things in order to accomplish your goals.
9. Impact happens through other people
More than anything else, a coordinated research program is about changing people's minds and behavior.
Whether you are creating a dataset, a tool, or a demo, you will need many other people to take action based on your work in order for it to be impactful.
For example, the goal of an ARPA program is to make a specific set of people realize that something that they previously thought was impossible, extremely unlikely, (or didn't think about it at all) is instead possible and something they should be working on. What that change looks like will depend heavily on your domain and the outcomes you're hoping to achieve (this is a big reason your job is hard!)
Figuring out what you need others to do based on your program is why the idea of a "theory of change" is particularly important.
10. If it doesn't 10x, it's not worth it
One of the core goals of a coordinated research program is to enable work that wouldn't happen in other organizations because of its high chance of failure. The other side of that coin is that if the program does succeed, it needs to create a reward that was worth that risk. There are plenty of organizations that will work on incremental improvements or small wins.
A heuristic for what makes a program's goals ambitious enough is that, if successful, it will create an "order of magnitude" improvement on how it's done today. In some cases, understanding what order-of-magnitude improvement looks like is straightforward: goals like reducing the size of an instrument or cost of a material by 10x or increasing the maximum power output of a system by 10x.
In other situations, "order of magnitude" improvement is fuzzier --- it needs to be a step-change, enabling things that were previously impossible: getting autonomous cars to finish a course that nobody could previously finish or networking together multiple computers from thousands of miles apart in a scalable way.
Throughout all of this, think about multiple timescales and priorities simultaneously
You need to think about making concrete personal progress on the timescale of a week, idea-and-people program progress on the timescale of months, technical program goals on the timescale of a year, and broader impacts on the timescale of a decade. At the same time, you need to consider all at once the incentives of researchers, ambitious but not impossible technical goals, how to make the work as general purpose as possible while still being pragmatic, and how the technology is going to get into the world.
The questions you should be obsessed with
-
What research will not happen under the current system? Some ways to break that question down is to ask what work is:
-
Too weird for most government funders?
-
Too engineering-heavy/not-novel/coordination-heavy for one or more labs to self-organize around it?
-
Too researchy/high-uncertainty for a startup?
-
Too unaligned with current paradigms for a big company to take it seriously?
-
-
If something would be incredible if it worked, why hasn't it been done yet? (With as much specificity as possible.)
-
Where are the weirdos? It's easy to gravitate to the people doing good work at top labs, but they are often well-funded already and good at pushing out their ideas. Think outside of the usual Big Cities. (Of course, often the best people are the best for clear reasons and sometimes they're still unable to work on something important).
-
What happens after this program? While we're not in the business of developing products, we are in the business of unlocking impactful technologies. In order for technologies to have an impact, they need to be carried forward by people whether that's in the form of a further research program (with its own theory of change), a nonprofit organization, a community using open source technology, a startup, companies incorporating it into their own product line, or something else. DARPA has a mixed record on transitioning in part because it rarely thinks about what happens after a program ends until the program is almost over.
-
What are fast clever experiments that could answer crux-like questions about risks or possibilities?
Identifying Who Cares
One of the hardest questions of The Heilmeier Catechism is the first half of question number four: Who cares? If you are successful, what difference will it make?
Answering "Who cares?" is tricky because answering it directly doesn't get at the idea behind the question that will help your program succeed. The direct answer is often along the lines of "a few researchers." Most people are not invested in things that they think are impossible, and if your program is genuinely trying to turn the impossible into the inevitable, most people beyond a few diehards will not care.
A more useful framing for this question is: "Who will care about the program's results if it was successful? And what difference will it make to them both short term and long-term?" Or put differently: "who will see the output of the program and think 'ah! That could be valuable to me' and ideally imagine a trajectory where it achieves that?"
Pragmatically, "who cares?" is about the program's output because the technology needs a home at the end of a program. The world is littered with the cold, stillborn bodies of promising technologies that nobody cared about enough to nurture until they could survive on their own. That home could be as humble as a nonprofit, a smattering of labs with funding from other organizations, or startups that still need to do a lot of work. But in order for even those things to happen, both people doing the work and people who will fund those homes need some promising evidence that they'll be able to achieve their goal. One of a program's jobs is to give them that evidence. "Who cares?" tells you who that evidence is for, and therefore what that evidence needs to be.
For better or worse, every program needs a narrative about why it is worth deploying scarce resources of time and money on: this is what will get it funded, align people who are working on it, and act as a discriminator on how to prioritize work. One of the most powerful narrative structures is: "if we can do X, it will remove bottleneck Y, which will enable Z."
One framing for a program is that "a particular group would do something drastically differently if these specific technical things were different." When we talk about "who cares," we're talking about that particular group.
Figuring out who cares is extremely hard! Answering that question well is a big chunk of program design.
Pragmatically, how do you figure out who cares?
In order to answer the question you need to talk to a lot of people. There are of course exceptions if you're building the thing for a broader group you're part of, but this situation is rare. Building a thing because you think it's cool or important is not building it yourself --- you need to be building it because the group will want to use it to do something they find valuable.
Before talking to anyone, you should have a hypothesis about a group and what they might find useful. (Eg. People who build membranes care about having better control over microscale pore structure). You shouldn't come out of the gate and ask about it directly -- be intensely curious about their context -- but it's important to have some anchor in your head to bias your line of questioning. Otherwise, conversations tend to stay incredibly abstract and not particularly useful.
Start by simply trying to understand what the constraints on their work are and what they care about. Ideally you lead them as little as possible, but you do need to give some framing about why you're asking questions --- eg. "I'm working on a program to explore X" and maybe "I have a hypothesis that Y." If you're lucky, they'll riff off of that, but most of the time they won't.
Some things to keep in mind:
-
These people often don't care how the technology you're working on works as long as it stays within their constraints.
-
You should never ask people "what do you want?" If you ask "what would you care about?" They will never give you a good answer, a la the supposed Henry Ford quote "if I asked people what they wanted, they would tell me 'a faster horse.' "
-
You should rarely even ask "would you care about <this specific program output>?" Because people won't tell you the truth: either they'll say yes to make you feel good or "no" because they have no imagination. You need to come at it sideways.
-
The trick is to iterate on hypotheses until you have something nuanced and precise that will stand up to scrutiny.
Note: this is secretly all startup customer discovery advice
Resources
Identifying Performers
Note that this writeup is ARPA-program specific
Without good performers, an ARPA program based on the best ideas in the world will fail. So take it seriously! Finding good performers requires a healthy mix of sales (seeking out and convincing great people to join the program) and marketing (sending up signal flares so that great people can and will seek you out).
Some specific tactics
-
Requests for Information (RFIs). RFIs are a standard way to open yourself to good inbounds. RFIs are one of the broadest nets you can cast, but it's important to note that they will bias towards people who are paying attention to whatever channels you're advertising the RFI on and have the time to write it up.
- It's easy to write an RFI but hard to write a good RFI that is both broad enough to solicit good ideas from left field, but not so broad that even good performers go off in some direction that is far from where you hope they would go. In order to do this, it's important to have done a lot of work beforehand to refine your ideas and understand how people commonly misinterpret them or go down not-helpful rabbit holes.
-
Pay attention to excitement levels. Whenever you're talking to potential performers (or anybody!) pay attention to how excited they are about the program: do they volunteer ideas and ask good questions or just try to convince you that what they're already doing is a good fit.
-
Go to conferences outside your field. Maximize the conference by reaching out to promising people beforehand, go to the hallway sessions instead of talks, and drop in on conversations. Pay attention to who engages when you tell them about your program.
-
Physically visiting labs. If there are people or groups that you think are particularly promising, visiting their labs can be incredibly informative even though you could do a phone or zoom call. Being in the lab has a whole slew of benefits:
-
It can give you a much better idea of their capabilities and how much else they have going on.
-
Seeing the lab can generate questions or ideas that wouldn't come up otherwise.
-
Being in the lab gives you a chance to interact not just with a PI or CEO, but the people who will actually be doing the work.
-
-
Paying attention to performers on previous programs. People who have worked as an ARPA program before (as grad students and postdocs in addition to as PIs) tend to understand the urgency and ambition that they're signing up for so people who have been on successful performer teams in the past are a good place to look for performers in the future.
-
Hold office hours. With modern technology, it's easy to hold "Digital office hours" where people can ask you questions and pitch you ideas.
-
Holding workshops. Once you've narrowed down your ideas and have a sense of who might be good performers, holding a workshop can help you learn more about their engagement and how they might interact with other potential performers.
Some things to note
-
Big-deal labs and organizations usually have stronger agendas so you need to do work to make sure that they're actually aligned with what you want to do.
-
More broadly, don't over-index on "badges": pedigrees, awards, or how reverently people in a field talk about them. Sometimes the best performers have a chip on their shoulder and come from a 2nd tier institution but are extremely qualified for a specific project.
-
Passion and competence aren't substitutes --- good performers need both.
-
Make sure you pay attention to a potential performer's ability to spin up quickly. While you may run into excited people with great ideas, you also need to judge them based on their ability to quickly turn money into work. Unfortunately, this means you often need to rule people out if they aren't PIs in labs or director level or above in companies. (If you can give them the ability to spin up quickly, by helping them start a lab or work in an existing one, this calculus changes.)
Be aware of your specific organization's rules around how you can solicit and engage with performers. The tactics you have at your disposal depend on the Institutional moves that are open to you at your particular organization. Eg. Some organizations require you to do an open call for any grant, contract, or position. Even in that situation you should (ideally, if permitted) identify who you would like to work with and make sure that they're aware of the call and encourage them to apply.
After you've identified good performers you need to make sure they are sold on the program's vision (even if they have come to you). See Selling a program.
Navigating a Network
A large part of designing a program involves navigating networks that you may not be part of and creating new ones.
What are the goals of these networks?
-
Finding potential collaborators -- employees, performers, or others
-
Finding potential "customers" for the program
-
Unearthing unexpected directions
-
Understanding what different communities think about your program
-
Identifying bottlenecks and key risks
Depending on what you are looking for, you'll want to talk to people in different positions:
-
If you are looking for external collaborators, aim to talk to decision makers, whether it's a PI of a lab or someone with equivalent authority in a company. It's easier to get in touch with postdocs/staff researchers and often they will be more excited and even have better ideas. However, to create performers you need to get people with decision making authority on board. (Of course excited non-decision makers can connect you to decision-makers.)
-
Similarly, if you are looking for potential "customers" you want to talk to decision makers who could affect the technology's ability to move forward.
-
If you are looking for bottlenecks and risks, you'll want to talk to a wide array of people who have been around a subject for a while. Most important bottlenecks and risks around technologies only make themselves apparent with sufficient context and experience. However, you don't want to take any one person's view on it because it's easy for single experiences to determine what someone thinks is feasible.
Whether or not you are part of a network primarily affects how you begin working your way through the network:
-
When you are already part of a network Start with the people you have the most social capital with --- people who you can ask dumb questions of and who are the most likely to be generative.
-
When you are not already part of a network Before diving into a network you're not part of, it's helpful to read some literature (blog posts, papers, parts of books) just to get an anchor point. These will probably be the wrong resources but it gives you a starting point for both people and questions. (Don't spend too long on the literature! It's the sort of thing you can spend infinite time on.) Once you have some initial questions, reach out to corresponding authors on papers that seem particularly relevant and go to conferences to just wander around and talk to people.
It will be tempting to talk to the highest-status people in a network --- they will be the most salient both from the literature and from people's recommendations. However, there's a strong correlation between individuals being high-status and having their own strong agendas.
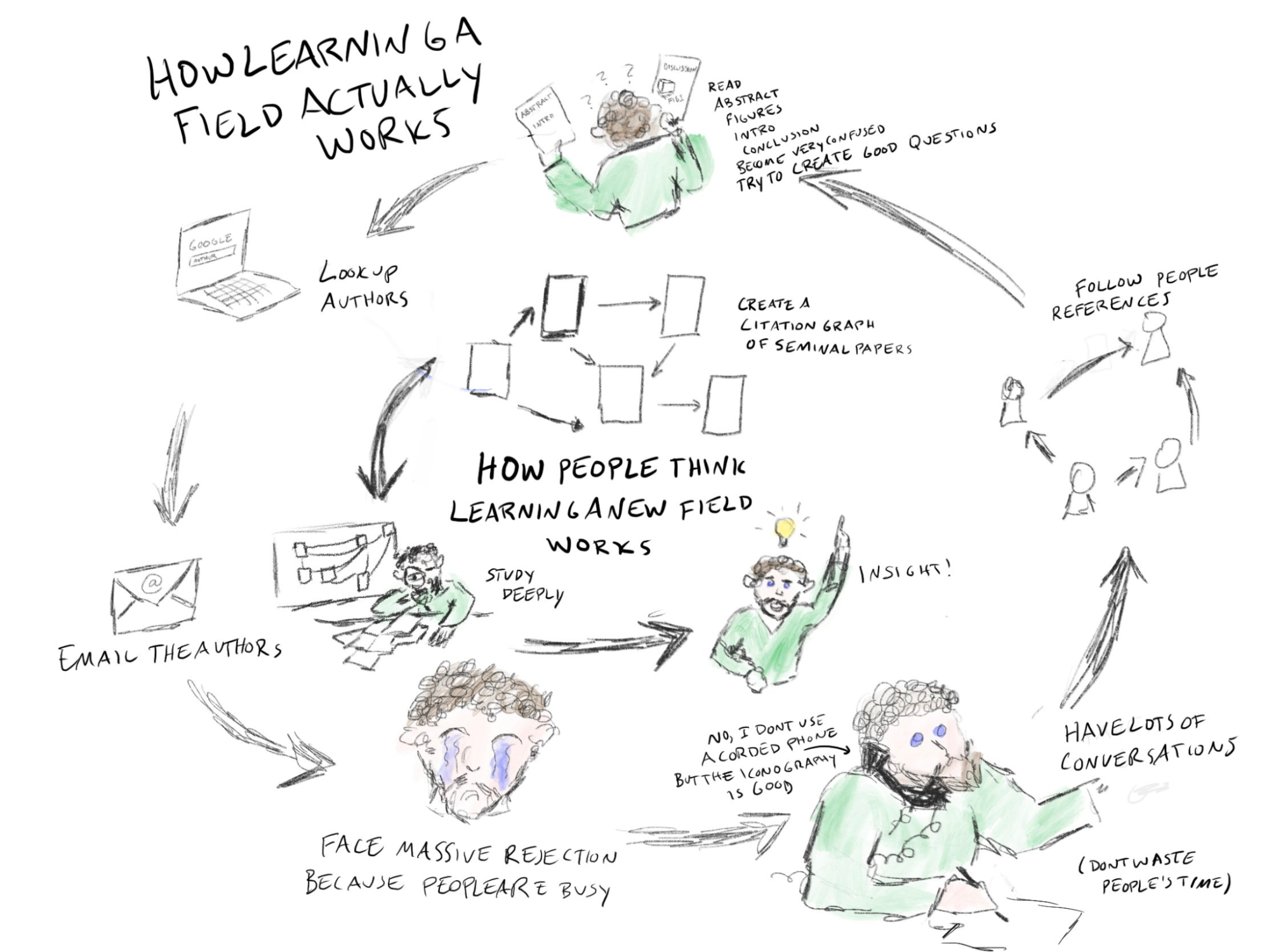
Keep in mind that you don't need to win over everybody --- you just need to find the people who are potential allies. Most people you will talk to won't be useful.
You do not need to do everything that everybody you talk to recommends --- this would take much more time than you have. There is a skill to sorting through which pointers --- people and resources --- are worth your time.
Something to watch out for is that it's easy to over-index on what academic researchers think is important and possible. There are several reasons for this: academics write the vast majority of papers, which are good starting points for digging into an area; they're generally more open to talking; and their emails are just easier to find! However, academic research priorities and challenges are often different from the ones you need to tackle to have a large impact (unless you're simply trying to advance an academic field.)
There are several concrete tactics that apply to navigating any network, regardless of whether you are part of it or not:
General Tactics
-
Always have some "hypotheses" that you're gathering evidence for or against as a way of directing your network navigation. These are hypotheses about your program -- what's important, specifics of what you should focus on, theories of change, etc.
-
Take advantage of LinkedIn's 2nd degree connection feature to figure out who you know who might know a person you're trying to talk to.
-
Reach out early, reach out often. You need to reach out to many more people than you think you do. For every good conversation, you will have many bad conversations and even more ignored emails.
-
Always follow up. Just because someone didn't respond, doesn't mean they don't want to talk to you. People are just busy. I generally follow up once/week until I've followed up three times or they have explicitly told me they're not interested.
-
Pay attention to institutional affiliations --- companies, universities, specific labs and teams --- and when people were at that institution to see whether they might be able to connect you to a target.
-
Pay attention to coauthors for the same reason.
-
Use some kind of software to track people. You will ideally be talking to far more people than you can keep in your head. This tracking software can be as simple as a spreadsheet. Other options include Airtable, Folk[2], Notion, Hubspot to name a few. None of these is the "best" -- the best tool is the one you'll use.
-
It's generally harder to talk to people at non-academic organizations (companies, the government, etc), but it's incredibly important! Some tactics for getting to folks:
-
Talk to former employees: they usually know people who are still there and may also be able to tell you the information you're looking for.
-
If you want to talk to a startup about their technology it's often more productive to talk to people who worked for a failed startup in the same space.
-
Organizations often have standard formats for their emails -- if you can figure that out (there are services online that help) you can cold email people.
-
Reaching out to people on Linkedin has a low hit rate but is worthwhile.
-
Email Tactics
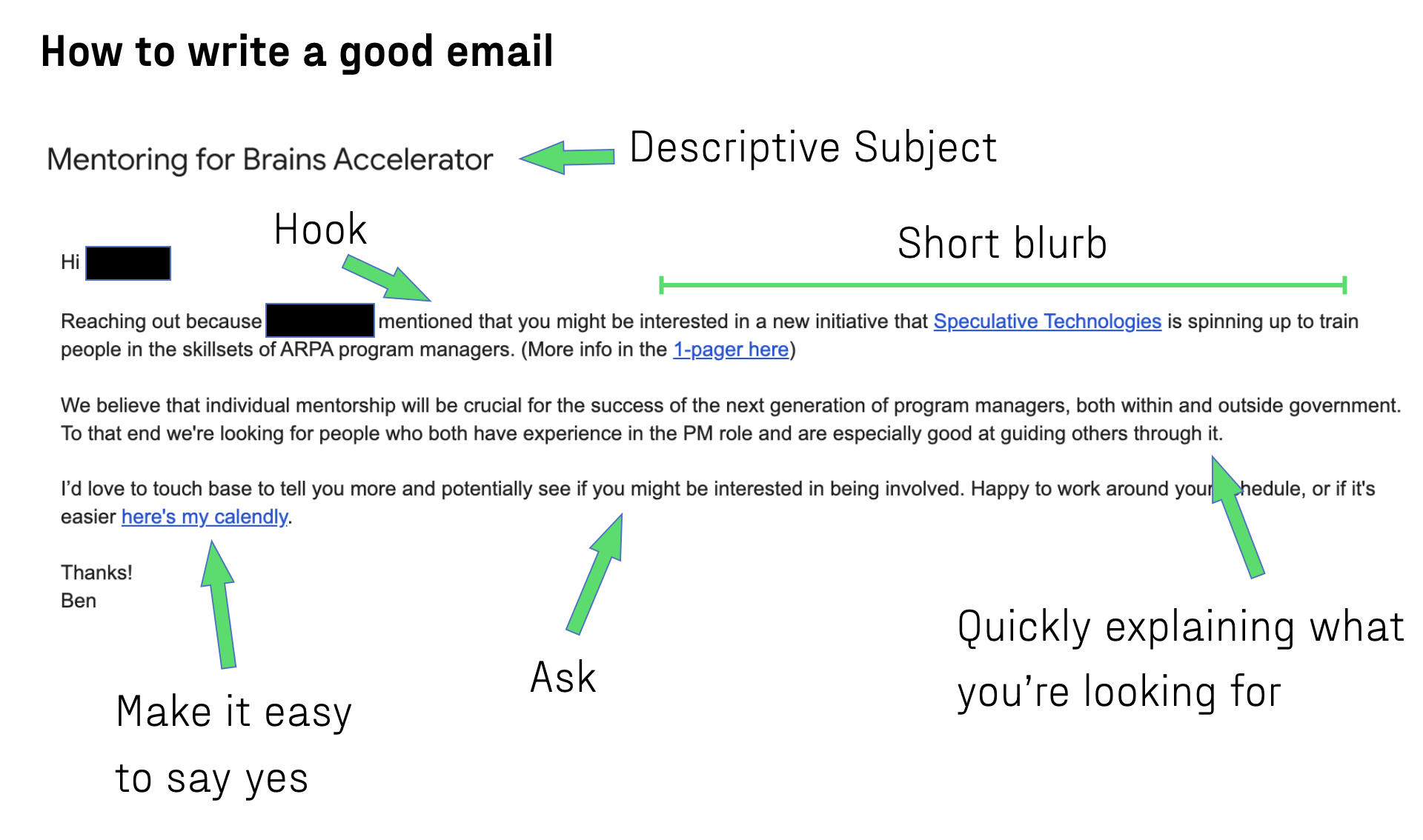
-
Having a good blurb about who you are and what you're doing is critical. A good blurb is short (at most three sentences) and gives the most important information up front. Ideally it's customized to your target's interest. (Here are some examples of blurbs).
-
Employ the double opt-in intro when possible (really do read this if you haven't heard the term before).
Conversation Tactics
-
Do some research on people before you talk to them: read their CV, skim the abstracts of their papers, look at their website. Doing your research both lets you ask much better questions and flatters people, making them more likely to help you.
-
While you do want to leave room for serendipity, it's important to have concrete questions going into every conversation. Most people will default to talking about higher levels of abstraction but lower ones are more useful. Additionally, concrete questions are better hooks to get people to talk to you in the first place. Ideally, people would understand what you're going for and summon ideas for other people you should talk to and useful ideas you should pursue.
-
In every conversation, make sure you ask "who else should I talk to?" (Ideally about specific things that came up in the conversation)
-
If they recommend people you should talk to, make sure to ask "can you make those introductions?"
-
Follow up conversations with a thank you, a short recap of what you talked about (in case you remembered something wrong or they have additional thoughts), and a list of the people who they are going to introduce you to.
Knowing when to stop --- you can do this kind of network exploration forever, but because you need extreme urgency and a bias towards action, at some point you need to decide that you've talked to enough people (of course you should continue talking to people, but it needs to become a back burner activity). Three ways to make this call are
-
Pay attention to your learning rate. When you notice that you tend to hear the same things in each conversation, it's not as necessary to have more conversations.
-
Pay attention to people-loops closing. When the people you're talking to tend to recommend talking to the same set of people that you've already talked to, you may have exhausted the experts in an area. (Of course there could be a disconnected cluster of people).
-
Time boxes. Give yourself clear deadlines to stop being in full conversation exploration mode. Frankly, this is probably the most straightforward and pragmatic approach.
What Ideas Make a Good Coordinated Research Program
Coordinated research programs can take many forms, but not all ideas -- even great research ideas -- make a good coordinated research program. It's impossible to enumerate the exact characteristics of ideas that make a good program: every characteristic would have many exceptions. (It doesn't help that the entire term is an umbrella term for a whole class of things which themselves are incredibly nebulous!)
The best we can do is a list of characteristics where the more of them an idea can check off, the more likely it is to make a good coordinated research program. We can then go one level deeper and list the characteristics that make an idea particularly well-suited for one type of program or another (eg. an ARPA program vs. an FRO).
Good coordinated research programs...
-
...have a clear goal. Unlike many good research programs, the best coordinated research programs don't muddle forward while supporting wide-ranging work, even if it's great work. In part this is because there are plenty of organizations that run broad research programs and in part because a diffuse program can't get much done during the few years that characterizes a coordinated research program if it is appropriately ambitious.
-
...are extremely ambitious. Another way of framing this is that they will be big if true. It's easy to imagine ambitious ideas, but much harder to create ambitious ideas that also have a clear goal that is feasibly achievable.
-
... have a clear argument for why that ambitious goal is possible. It's easy to lay out an ambitious goal, but hard to lay out clear ambitious goals with clear reasons why it might be possible to hit.
-
... can imaginably get to that ambitious goal in ~five years. A few years is shockingly short for ambitious research, so ideas need to walk a fine line. (There is no structural reason for this five-year timeline, but acting on a much shorter or longer timeline creates a cascade that makes the idea into a very different thing.)
-
... require more than $1M but less than ~$50M to hit those goals. The type of work you need to do to plan and fund work on both the low and high end becomes very different.
-
... have some number of people who think that goal might be impossible --- and they might be right. Not only is this a good heuristic for ambition, but because one of the most useful things a program can do is to turn the impossible into inevitable, it's important to have a clear set of people whose minds can be changed.
-
... focus on neglected problems or very different approaches to existing problems. Coordinated research programs are relatively small in the grand scheme of money spent on research worldwide. On the margin, a program working using a slightly different approach to tackle a problem that many other people are working on will not have much impact.
-
... have a clear theory of change. At the end of the day, a coordinated research program can create amazing knowledge or technical capabilities, but it won't matter unless other individuals or organizations carry it forward after the program ends. In order for that to happen, the program needs a clear idea (that can of course change over time) of what happens when the program ends and how to enable that. (Even though many times the impact doesn't happen the way the theory of change intended!)
What ideas should be a specific type of coordinated research program?
ARPA programs and Focused Research Organizations (FROs) are two of the most legible structures for coordinated research programs, so it's worth digging into specific characteristics that make an idea particularly well-suited for ARPA programs or FROs.
For the purpose of this discussion, "ARPA program" is shorthand for external research coordinated by an empowered program leader, while "FRO" is shorthand for a time-boxed nonprofit research that primarily does internal research. In reality, "ARPA programs" and "FROS" are nebulous concepts that don't have a clean line between them. For more on the difference between FROs and ARPA programs, you can also read this piece.
It's easier to start ARPA programs and FROs because of their institutional legibility but keep in mind that coordinated research programs can take other forms, most of which do not have names!
What ideas make a good ARPA program?
Good ARPA programs...
-
... have work that can be parallelized. Mechanically, ARPA programs consist of a number of different performers working on different projects. This style of work lends itself best to work that can be parallelized, whether it's different components of a system or different approaches to a problem.
-
...require expertise that lives in existing organizations and would be hard to hire away. Externalized research has the advantage that it doesn't require researchers to leave their home institution.
-
... have several possible routes to impact. A common theme of ARPA programs is that they make a number of bets not just on approaches but on the way that they're going to have an impact: the technology is potentially taken up by a large organization or a startup; or maybe it starts a new field of research.
Some examples of good ARPA programs (and why):
-
ARPANET. The ARPANET program created the network that would eventually become the internet. It was well-suited to be an ARPA program because:
-
It was justified in terms of an immediate need (robust communication infrastructure) but had the potential to go on to be much more impactful.
-
It involved both grungy engineering problems (building and installing the hardware) and hard research problems (coming up with robust protocols).
-
It required coordinating a number of different organizations -- contractors and academic labs -- with different expertise.
-
-
DARPA Grand Challenge. The DARPA Grand Challenge was a prize competition for a vehicle to autonomously navigate across the California desert. It was well-suited to be an ARPA program because:
-
It required unconventional funding mechanisms. It was one of the first times the US government offered prizes for a competition.
-
Autonomous driving was a technology that had been worked on for decades but was at the point where a strong push could create a step-change in its capabilities.
-
It shifted perception of autonomous vehicles from something that might never happen to something that was inevitable, leading to tons of follow-on work in large companies and startups.
-
-
GPS miniaturization. The original GPS receivers used analog signals and weighed 50 pounds. The MGR program created GPS receivers that were the size of a pack of cards and digitized the system. It was well-suited to be an ARPA program because:
-
It was impactful work that wasn't particularly "novel" (GPS already existed, the challenge was just to make it smaller).
-
It had a clear but incredibly ambitious goal.
-
It opened the path for many subsequent improvements.
-
What ideas make a good FRO?
Good FROs...
-
... have a clear goal from day one: whether it's a dataset, a well defined tool, or something else.
-
... know precisely how they'll deploy ~$50M early on in the program's lifetime. This is harder than you think!
-
... have a single project or tight coupling between projects from day one.
-
... have strong conviction about the right approach at the project level early on.
-
... have a core team who are willing to work full-time lined up before starting. An FRO needs to hit the ground running and is initially judged on its core team.
Some examples of good FROs (and why):
-
E11 Bio. E11 Bio is mapping the entire mouse brain at the cellular level. The organization is creating a dataset and developing technologies that are primarily useful for researchers -- a valuable public resource that would be incredibly hard to monetize. It is well suited for an FRO because:
-
Doing this brain mapping requires a lot of engineering to extend existing technologies and a lot of repetitive but expensive work to slice brains, scan those slices, and analyze them.
-
While all of this work is hard and requires a lot of problem solving, it could be described at a pretty granular level early on.
-
-
Cultivarium. Cultivarium creates open-source tools to enable scientists to work with novel microorganisms: things like data on growth rates, protocols for modifying their DNA, computational tools, etc. It is well suited for an FRO because:
- There's a vaguely standard set of tools and data you want to create for each microorganism; it just takes a lot of work to create them.
-
RAND Health Insurance Experiment. The RAND corporation had a lot of clear experimental questions that could only be tested in the context of a health insurance company. So they started their own. While the concept of an FRO had not been invented yet, it was well suited for an FRO because:
-
It was a time-boxed experiment (most of the work was done in the first five years).
-
It was answering clear questions.
-
It was clear what execution looked like from day one.
-
What ideas aren't good fits for coordinated research programs?
Some examples of good ideas that are a poor fit for a coordinated research program.
-
LIGO. The Laser Interferometer Gravitational Wave Observatory is a large-scale physics experiment and observatory designed to detect cosmic gravitational waves. It has been incredibly impactful for our knowledge of the universe but would have been a poor coordinated research program for several reasons:
-
LIGO took several decades of prototyping and building.Coordinated research programs generally exist on shorter timescales.
-
LIGO took hundreds of millions of dollars to get to the point where it could take observations. Coordinated research programs are generally tens of millions of dollars.
-
LIGO's main focus is expanding the scope of human knowledge rather than creating new capabilities.
-
-
SpaceX. While it involved a lot of high-risk technology development, SpaceX both had a clear path to profitability and the work to create that technology was best done in a single, tightly-coordinated organization.
How do I operationalize all this?
You can operationalize these characteristics by creating a checklist and making sure that your program idea can tick off all the boxes (or you have a very precise way to get around that requirement).
Identifying Bottlenecks and Key Risks
There are an infinite number of things one can do to advance ambitious technology. Identifying bottlenecks and key risks is a powerful way of both cutting through the noise to prioritize work and also to sell ideas to stakeholders.
Bottlenecks and key risks are nebulous concepts: neither has hard boundaries that define what does or does not "count." To give rough definitions:
-
Bottlenecks are the problems/capabilities that, if solved/created by a program, would enable many other people and organizations to move forward on the technology you care about.
-
Key Risks are potential facts about the world that, if true, would make your program's approach infeasible.
Some heuristics for how to conceptualize bottlenecks and key risks:
-
Bottlenecks are things that a program can address, while key risks are potential game-stoppers internal to a program.
-
Bottlenecks are the facts that make a technology feel intractable or hopeless.
-
Bottlenecks are the rate-limiters on progress in an area. Technical progress is much more abstract than manufacturing, but the analogy to a factory where one machine can process parts much more slowly than all the others can be useful.
-
Technology bottlenecks usually take the form "Some group, A, would do B if C were different." C is the bottleneck.
-
Key risks are often the reason that other people think what a program is trying to do is impossible.
-
Key risks are often the black box that you want to say "we'll figure that out later."
Some examples of technological bottlenecks and key risks:
-
One bottleneck to cheap spaceflight is reusable launch systems. Some key risks for creating reusable launch systems are rocket engines that can restart consistently and control systems to land a rocket successfully.
-
One bottleneck to rapidly developable vaccines was a mechanism for creating specific antigens on demand. A key risk for RNA vaccines to address this bottleneck was a mechanism of encapsulating the RNA.
At the end of the day you need to drill down to the specific bottlenecks your program can address and the key risks that can be addressed by individual projects within your program*.* It's straightforward to say something like "cost is bottlenecking carbon removal" but drilling down into actionable, technology-based drivers of those costs is hard.
There are two ways of dealing with bottlenecks: removing them or going around them. Removing a bottleneck looks like shrinking a critical system from the size of a shipping container to the size of a briefcase. Going around a bottleneck looks like removing the need for that system all together.
When you are designing your program, it's critical to address the key risks (this is another framing for the idea of "failing fast." See: Tackle the monkey first
Brass Tacks and Tactics
Identifying the bottlenecks and key risks (especially to an actionable level) is not straightforward.
People don't often think in terms of bottlenecks and risks, especially at the level of a whole technology instead of their own personal projects. As a result, you can't just read the answer in literature or ask people "what are the bottlenecks in your area?"
Here are some tactics for teasing out key risks and bottlenecks:
-
Drawing block diagrams and fishbone diagrams can help identify the causal connections in a system and enable you to trace a high-level bottleneck to an actionable source.
-
Sankey diagrams can help identify what the biggest contributors to some flow is (eg. Identifying why something is expensive).
-
Fermi estimates and breaking down the physics behind a system are helpful for identifying bottlenecks and key risks: "if we were to build this at an impactful scale, it would <require all the niobium in the world><cook a human brain with the amount of energy it puts in> etc."
-
When you are talking to experts in an area who don't think that an idea will work, dig into why they don't think it will work. These are likely to be some of the key risks: if the experts are right, the program is dead and the work is to prove them wrong.
-
When you're talking to potential "customers" for a program (see Identifying who cares), try to figure out what subtle things would cause them to dismiss the program's outputs (does it need to fit into a briefcase? Will nobody use it if it's above $100?). These are also key risks.
As an example of a good bottleneck analysis, see Adam Marblestone's positional chemistry bottleneck analysis.]
Planning for Transition
In order for a program to have an impact, it needs to transition beyond the program. "Transition" can mean many things: continuing as an active area of research in other organizations; being incorporated into different products; spawning one or more startups; becoming an open source project; and many others.
Failure to transition is how many programs fail to have impact, even if they hit their technical goals.
Design decisions early in a technology's lifetime have profound effects on its ability to be something that somebody wants and survive beyond the R&D cocoon. Small differences, like the ability for a new material to be created using existing equipment or interface with standard connection points, can have a huge impact on its ability to transition.
Figuring out what these differences need to be depends both on a strong hypothesis about what is going to happen at the end of the program and a deep understanding of what the technology actually needs to do in that situation.
Furthermore, a technology's ability to transition is sometimes a function of long preparation for multiple parties. A team of researchers who reach the end of a project and say "ok! Let's become a startup now!" will often fail. A big organization needs time to wrap their head around a new technology (especially if it is a different paradigm) and often wants specific proof-points that aren't obvious or derivable. Even if it's something that would drastically help their business, you often can't just show up with a brand-new technology and expect them to absorb it with open arms.
Concrete tactics
Note that many of these will vary depending on your specific program and organization
-
Spend a lot of time building relationships with decision-makers at organizations who might provide homes or support for our technologies after programs end: these could range from large companies to government organizations to large foundations. Understand what problems or constraints these people are facing that your programs could potentially solve or remove.
-
Ask "Who will care about this program if it hits its goals? What does the technology need to look like in order for them to care? What needs to happen in order for it to look that way? What happens at the end of the program?"
-
Continually update your transition hypothesis throughout the course of a program.
-
Find a business-minded CEO and get them to start working with a team for a year before the program ends.
-
Help a team spin up a demonstration manufacturing line.
-
Negotiate with a large foundation to fund a spinout nonprofit.
-
Coaching people building the technology on how to start thinking like entrepreneurs.
-
Create self-reinforcing networks of researchers and stakeholders through conferences, publications, etc.
-
Have a large company as a performer whose funding is contingent on spinning up a new product line around the program's technology.
-
Make sure that the IP created by the program doesn't become locked up in a university tech transfer office or large company.
-
Get teams to start talking to potential follow-on funders long before the program ends.
-
Talk to other funders across government, investors, and foundations about what they would want to see in order to support the technology after the program ends.
Related resources
-
Loonshots --- specifically the section around "Manage the Transfer not the technology"
Program Failure Modes
Sufficiently ambitious programs can't eliminate the chance of failure. However, you can affect the odds that you fail in a new way due to an unknown unknown rather than fall into the common traps for coordinated research programs.
It's worth unpacking a bit what it means for a coordinated research program to succeed or fail. Coordinated research programs are not like businesses that are judged on their financial success at the end of the day regardless of whether they achieved that success through a brilliant invention or driving competitors out of business (often it is both!) In a narrow sense, a coordinated research program is successful when it hits some set of technical goals. But in a broad sense, a program is successful when it has a large counterfactual impact: looking backwards, the world is very different (in a good, technical way) than if the program had never happened. Some examples of programs that were technical failures but counterfactually impactful include Have Blue that failed to create a flight-worthy plane but was pivotal for stealth technology or the first DARPA Grand Challenge, where not a single car finished the race but the teams and subsequent programs it spawned went on to create the entire autonomous car industry.
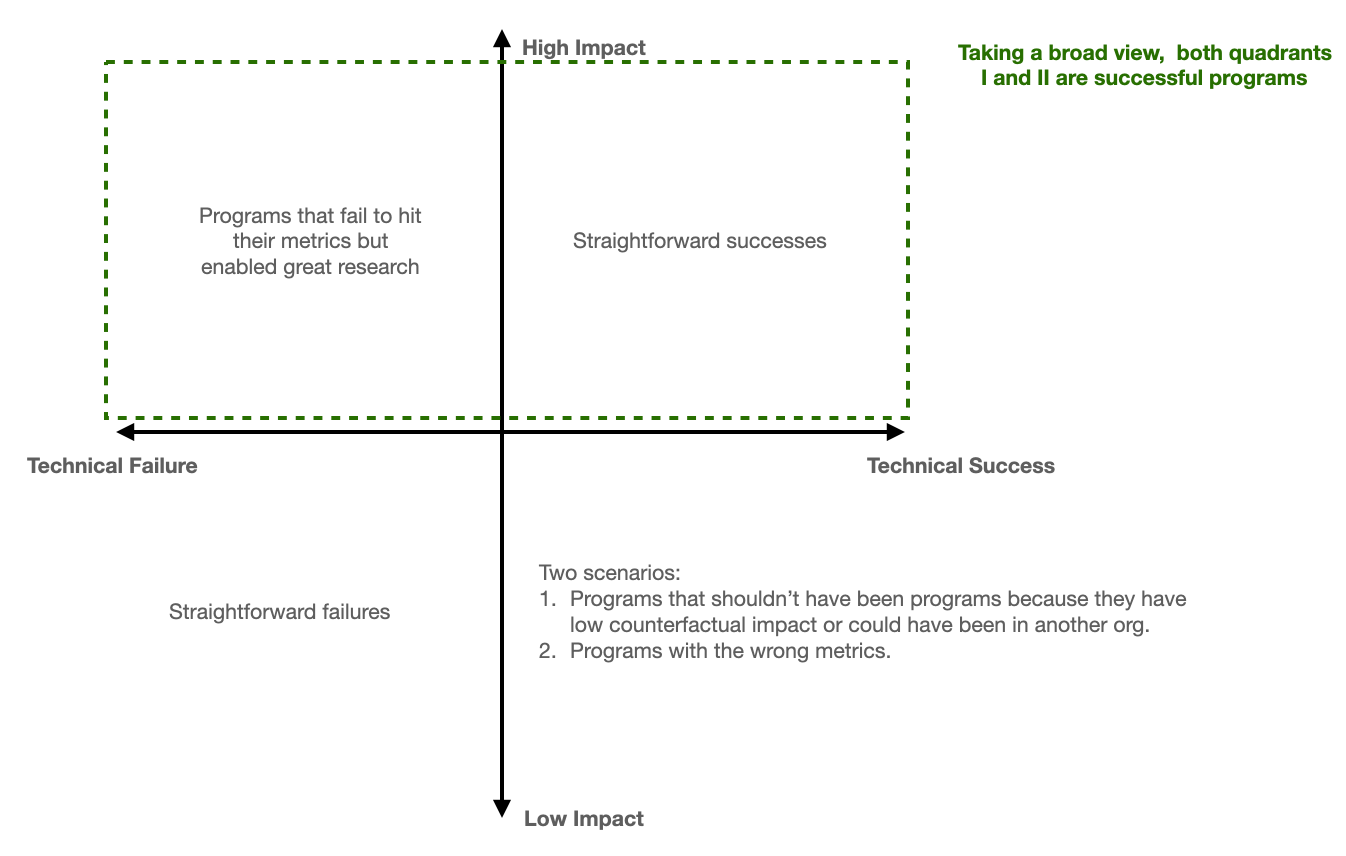
Ideally programs are successful in both the narrow and broad sense: it's much harder to know whether a technically failed program will, or has had, a counterfactual impact. It's harder to sell subsequent programs based on technical failure that arguably had a counterfactual impact --- that impact usually takes years or decades to become obvious and counterfactuals are notoriously hard.
Failure modes are therefore things that can prevent programs from succeeding in both the narrow and broad sense.
There are an infinite number of ways that programs can fail, but below are some common failure patterns ordered roughly by where in a program's lifetime the failure occurs:
-
The program never gets off the ground or is canceled because management doesn't see the program's goals as within scope of the organization. One example of this was a program to create disaster response robots at DARPA that wasn't seen as directly relevant to defense.
-
A program leader listens to experts who say that something is impossible and abandons an idea or scopes it down, only to have another group succeed at the ambitious vision. DARPA ran many AI programs over the past several decades, but most of the AI breakthroughs did not come from these programs because the programs focused on improving existing paradigms instead of previously written-off methods like neural networks or left-field ideas like transformers.
-
Not asking the right questions about what is or is not possible. It's not an ARPA program, but Bell Labs' failure to create fiber-optic cables is illustrative: researchers asked "how transparent is the best glass?" The answer indicated that it is impossible to transmit light through a kilometer of glass. Charles Kao asked "how transparent could you possibly make glass?" That answer meant that light could travel through several kilometers of glass without amplification. As a result, Bell Labs wasted 20 years working on hollow waveguides instead.
-
The program's idea isn't compelling to management for one of many reasons.
-
An authority above management decides that the program is not a good use of money.
-
An approach is physically impossible.
-
An approach is bottlenecked by another technology that is not yet good enough.
-
The work ends up being far more expensive than expected.
-
The program leader who championed the program leaves and the replacement has a different vision or approach that confuses or alienates employees or collaborators.
-
Conflicts between the program manager and employees or collaborators.
-
The program primarily supports work that would have happened without it.
-
Technology developments outside the program make the program's output obsolete.
-
A solution doesn't scale (What does it mean for a technology to scale?)
-
A problem is primarily political or cultural instead of technical.
-
Regulations prevent the technology from getting out into the world.
-
Failure to transition because it disrupts an existing paradigm in the organizations that were expected to carry it forward.
-
Failure to transition because several different external collaborators worked on different components of a system and there's no incentive to put them together at the end of a program
-
(Related to the previous) IP from different projects gets locked up --- either in a single large organization that has no incentive to commercialize the work or in several organizations that prevent integration.
There's no foolproof way to avoid these failures, but some concrete tactics include:
-
Being brutally honest with yourself about the biggest risks to the program (see Identifying bottlenecks and key risks) and addressing them up front.
-
Frequently check in with yourself on whether your program is showing signs of a potential failure mode.
-
Be open to feedback on whether you are trending towards a specific failure mode.
-
Divide a piece of paper or whiteboard into three columns.
-
In the first column, write down as many ways that the program could fail as you can think of. Be realistic and specific!
-
In the second column write down what actions you could take during the program to minimize that chance of failure. There may be nothing you can do besides run straight towards it! These are the key risks that you should get to as quickly as possible.
-
In the third column, write down what indicators you would have that you were headed towards a specific failure mode. It's tough to accept, but if you are honest with yourself, these are some of your indicators of when you should think about killing an approach or entire program. (See Knowing when to quit.)
-
-
Bias towards failing by being too ambitious! The point of coordinated research program is to swing for the fences. One way to do this is to ask yourself "will I be proud to have failed trying to do this thing?"
External resources
-
Why does DARPA work? Section on DARPA Transition Failures.
-
Arthur C. Clarke's "Failures of Imagination and Failures of Nerve." From Profiles of the Future:
-
"Failure of Nerve occurs when even given all the relevant facts the would-be prophet cannot see that they point to an inescapable conclusion."
-
"Failure of Imagination arises when all the available facts are appreciated and marshaled correctly -- but when the really vital facts are still undiscovered, and the possibility of their existence is not admitted."
-
Mapping Incentives
At its core, a successful research program enables work that would not have otherwise happened. In order for this to happen, you need to give people the resources to do things they already want to do or you need to shift what they want to do. In either of those cases you need to think about people's incentives: what is driving them to act the way they do.
Incentives matter for coordinated research programs both so that you can effectively coordinate people within the program and also so that the program itself can be impactful. If you don't consider incentives, you are going to find yourself constantly frustrated by people who say they will do one thing and wind up doing another or simply won't cooperate at all. Furthermore, many programs attempt to address systemic problems. Most people are not malicious or stupid --- they're simply acting according to incentives you might not see. In order to make headway on these problems, you need to figure out what is incentivizing people in that system so that you can shift those incentives.
For example, companies are incentivized to maximize profits (shocking!) so they're incredibly unlikely to use a replacement technology that is [cooler/more sustainable/etc] if it costs drastically more than what they're currently using. Installing new technology also costs money so companies are rarely incentivized to use technologies that are small improvements over what they already use. Governments (and especially militaries) have different incentives such as keeping soldiers and citizens alive, making politicians look good, and appeasing lobbyists, so they are sometimes willing to pay significantly more for marginal improvements in capabilities.
There is no best-practice methodology to mapping incentives or incorporating them into your program design. One approach is long-form writing where you work backwards from the outcome that you want, describing who you want to do what and what their current incentives are. Another is to set up a spreadsheet or piece of paper with several columns:
-
Actor
-
Current incentives
-
Desired action
-
Why their current incentives prevent them from taking the desired action
-
Potential actions you could take to bridge that gap.
Figuring out what people's and group's incentives are in the first place is not straightforward. You can't ask directly both because it's incredibly awkward to ask "so, beyond the nice-sounding reasons, why are you really doing this?" and people often don't notice what's really driving their behavior.
Some tactics for discerning incentives:
-
Figure out what gets people in a particular position promoted or fired. Reaper functions dominate other incentives
-
Look at where an organization's free cash flow comes from.
-
Look at what someone's peers celebrate or condemn.
-
Look at whether an individual or organization is under any particular legal obligation.
-
Observe the patterns in trajectories and failure modes of people/organizations/ideas that look similar to people/organizations/ideas that you're considering.
There are also many questions that you can ask to tease out different sets of incentives. These questions are domain specific. Some of them include:
-
For academics
-
What stage of their career are they at?
- If they have tenure
-
How large a lab do they need to support?
-
What projects do they already have going?
-
What ideas have they pinned their career to?
-
-
For universities
- How could being involved in a program increase their prestige?
-
For large companies
-
What is their core product/revenue stream?
-
How is that product priced?
-
-
For people at companies
- Is this person's department a cost center or a profit center?
-
For startups
-
What are they trying to build?
-
What ideas have they sold to their investors?
-
How soon do they need to raise another round?
-
Once you understand the incentives at play, you can either try to shift them or work around them.
Ways that you can shift incentives:
-
Pay someone to do something
-
Create a field that they can publish into
-
Make them feel like their peers' opinions have shifted or help them find new peers
-
Change the opinion of their bosses
-
Convince them that a problem is actually interesting
Ways to work around incentives:
-
Enable someone to do more of what they want to do in exchange for spending some of their time doing what you want to do.
-
Convince them that what you want them to do is related to what they already find interesting
-
Shift your goals to be more aligned with theirs
Related
Selling a program
It's easy to think that your ideas should speak for themselves or that sufficiently incredible results will cause the world to beat a pathway to your door. Unfortunately, this is not the case! A program leader needs to be a salesperson from before their program starts until the day it ends or someone else takes over.
There are three major groups you need to sell a program to:
-
Management. This is a catch-all term for whomever has the authority to authorize resources for the program or shut them off.
-
Collaborators. These are the people who are going to be doing work during the course of the program.
-
Transition partners. These are the people who will carry on the work after the end of the program. Remember, technology is people.
Many tactics matter regardless of who you're selling to. Note that many of these may feel like they're in tension --- good sales is walking a tightrope:
-
Focus on the bottom line up front. Even if someone will want to get in the weeds, you need to anchor them on why they should care in the first place. This looks like saying something like "Our goal is to create price-competitive complex carbon-based molecules from atmospheric carbon instead of petroleum. Specifically, this program is focused on using cell-derived enzymes in a continuous-flow process." instead of launching into "there's a process where you pull the enzymes out of a cell and can then use them to do the same metabolic processes as in a cell ..."
-
Be precise. It is far more compelling to say "Our goal is to increase the number of probes that can be inserted into the human brain by two orders of magnitude without increasing price or damage" than to say "Our goal is to radically transform brain-computer interfaces"
-
Make the outcome of the program emotionally compelling. Even deeply technical people make decisions based on whether they're excited, scared, etc --- they're just better at justifying it to themselves. The tricky bit is that different people will find different facts, arguments, and styles of presentation emotionally compelling.
-
Spreadsheets are some of the best sales tools. It is incredibly compelling if you can show "here are the numbers that combine to show how it is today, here are the numbers around how it could be, and here are the numbers that show that this idea is physically possible." (This is also why Fermi Estimates are compelling.)
-
Practice your opening lines and answers to common questions. It's not just about what you say but how you say it. If you can respond smoothly and confidently, it will go a long way towards hooking your listener.
-
ABS: Always Be Selling. Try to get everyone you talk to excited about your program, regardless of whether you think it is relevant. The way you figure out how to sell an idea is through iteration.
-
After (ideally) every conversation, write down what questions people asked, where they seemed to be confused, and how you would have done it better (explained it differently, talked less, etc).
-
Understand who the decision maker is, and who the decision maker listens to. Realize that nothing matters until the decision maker is convinced, but part of that process is convincing the people the decision maker listens to. Ideally, get the person who the decision maker listens to, to iterate with you and explain the ins and outs of the system.
-
It's helpful to always have a 1-2 sentence blurb, a 1-2 page document, and a few slides available about your ideas, regardless of the format that you'll be pitching in. Don't wait until the idea is "ready" to create these.
-
If you can, get materials from people who have previously been successful with the person or group you're dealing with.
-
Pay attention to incentives!
-
Always make it clear how supporting your program will help whomever you're talking to achieve their own personal goals.
-
Help people feel like an idea was their own idea (even if it was yours.)
-
Do not underestimate the power of good plots and figures. It's time consuming, but often a good figure or plot can hammer home a point far more effectively than words. Adobe Illustrator and ipython/matlab are tools worth being familiar with.
-
People rarely act in isolation. Give people the tools to sell the idea to others: very clear logical lines of argument, numbers, or figures.
Some specific advice for selling your program to different groups:
Management
-
Pay attention to whomever management reports to and what they care about. Decision-makers ultimately need to justify their decisions to other decision-makers, peers, or the public.
-
Keep management in the loop as your ideas evolve. It's easier to sell an idea if it doesn't seem like it came out of nowhere.
-
Try to understand the decision-making process as well as possible: who will management consult, what materials do they want to see, etc.
Collaborators
- Make it clear how working on your program is aligned with their personal or career goals.
Transition Partners
-
Try to figure out what constraints they're under. If the program doesn't address or work within those constraints, it will go nowhere regardless of how promising results are.
-
Start the sales process to transition partners long before the end of the program.
Budgeting for a coordinated research program
There is no algorithm for figuring out a program’s budget. As such, most of the advice here will be frustratingly common sense. The unsatisfying reality is that budgeting for large programs is essentially a bundle of tricks that you use to come up with numbers that everybody involved thinks are good enough as opposed to an actual procedure.
At a high level, the budgeting process is some mixture of
- Bottom-up budgeting. Bottom-up budgeting is figuring out how much things will cost from “first principles” — figuring out how much equipment, consumables, person-hours the program will take and how much you expect each of those to cost.
- Comparables. Sometimes the most straightforward way to know how much something will cost is to compare it to what something similar cost in the past and make adjustments based on the difference.
- Top-down budgeting. In reality, your budget should be informed in part by asking “how much can we do with the amount of money we think we can get?”
To many technical people, comparable and top-down budgeting probably feel unsatisfying, but the uncertainty associated with any research program means that a bottom-up budget can be just as inaccurate as a comparison or a top-down budget. The most accurate budget will likely come from a mixture of the three. That scenario could look like “there’s a huge gap between the bottom-up budget and how much a comparable thing cost in the past, which made me realize I wasn’t accounting for a delay in this key part so to make it within the budget my organization normally authorizes, I need to cut this other project and redirect its funds towards making sure that part comes on time.”
The closest thing to an “algorithm” for budgeting a coordinated research program might be the following:
- Start with the Gantt chart. Any considerations about budget are downstream of the actual work that needs to happen in the program. You should have a good idea of:
- The different lines of work or projects that need to happen
- The milestones those projects will need to hit
- The dependencies between those projects
- Identify monetary bottlenecks. Most programs will have some places where more money won’t make things go any faster — things like clinical trials or regulatory approval processes that can’t be parallelized. It’s important to identify these places because in order to hit your goals on time, you may need to budget more for areas where money could buy speed. (See more below about the nuances of buying speed.)
- Understand comparables. Do enough research and talk to enough people that you have a sense of roughly the cost of both individual parts of your program (salaries, equipment, consumables, bureaucracy, etc) and the program overall. (This is yet another reason why navigating a network is important!)
- Put yourself in the shoes of the project leader. For any given project, put yourself in the shoes of that project's leader (this could be you!) and sketch out what you would need to hit your milestone and how much those things would cost.
- Write down your biggest assumptions. For every non-obvious number you write down, you should write down the three biggest assumptions behind that number. For example “I’m assuming that it will be much easier to do tests 2-5 after they figure out how to do test 1.” Bounce these assumptions off of people!
- Work backwards from different budgets What do you think you could achieve with $2M? $5M? $25M? Would that be compelling?
The massive caveat here is that the amount of time you should spend on each of these steps will vary greatly for different programs, organizations, and contexts.
There are four things to lean on when you’re thinking about budgets:
- Your own experience. In your experience, how much do projects like the ones you’re thinking about cost? How long do they take?
- (Ideally unbiased) technical experts. People who have seen projects that have a similar institutional structure to your program and the projects you’re proposing. In an ARPA organization these are SETAs or the equivalent (PSpecs and TSpecs in the case of ARIA)
- LLMs like GPT4 or Anthropic’s Claude even in early 2024 can be helpful for structuring assumptions and digging up comparable programs and budgets. The two caveats are to always double check numbers with a more trustworthy source and don’t ask them about “how do I budget a program?” directly or they’ll likely give you generic drivel.
- The business model of whatever organization you’re getting money from. (More on this consideration in the top-down budgeting section). This consideration goes for whether you’re an employee of an organization that runs programs like ARIA or are raising money from an external entity.
The rest of this section is primarily a bag of tricks and considerations.
Things to think about
- Consider overhead in your budgeting, both for yourself (travel, collaboration, operations if you need that) and for external collaborators if you have those.
- If you are going to be working with geographically distributed people, make sure you carve out a coordination budget to bring them together.
- Make sure you carve out a travel budget.
- When you’re thinking about how many people a specific project or task will take, there’s never a hard number. Instead you could think of additional people as making your timelines and chances of success more robust. It’s always possible that a single grad student could successfully hit all of a project’s milestones on time through superhuman effort or luck. However, the probability of that is low. More people on a project generally increases that probability. Adding more people will rarely decrease timelines in a straightforward way.
- You can often “pay more for speed.” There are straightforward ways to buy speed: purchasing a piece of equipment instead of building it, buying tools instead of using shared ones, hiring a specialist instead of having a grad student do it. You can also buy “stochastic speed” by parallelizing a problem: if each person or group has some probability of figuring it out each month, statistically the program will figure it out faster if you have more people working on the problem. For example, you could hire multiple contract research organizations to do the same thing to increase the likelihood that one will do it right the first time. (Just because you’ve paid someone to do something doesn’t mean they’ll do it the way you want and on time!).
- Scale is expensive! To some extent, your program’s budget depends on what scale you expect a solution to get to. (The question of scale feeds back from budgeting into program design: to some extent you need to tune your scale ambitions to the budget you believe you can command.)
- If you’re working with external collaborators, there are many different archetypes of projects, each with its own cost structures and amount of padding that needs to be built in. Some archetypes include:
- A large industry partner with academic subcontractors
- An academic partner with their own subcontractors
- Small companies
- If possible, keep a pool of money held in reserve. A rule of thumb is that this reserve should be 20% of the program’s budget. It has a number of uses, including:
- Unexpected expenses causing a promising project to run out of money
- Doubling down on projects that actually can absorb more money
- Opportunistically pursuing something that didn’t come up until you started the program
- Enabling transition at the end of the program: whether it’s seeding a spinout, matching a budget from a large company, or paying for engineering work to make a tool or dataset more usable.
- Run your numbers by people. Budgeting is a situation where the ~wisdom of crowds~ can actually work. (This is yet another reason why it’s important to build a network!)
- Different domains have different “budget physics.” Especially hardware vs software vs life sciences.
- Different organizations will have different rules about how earmarked your budget needs to be.
- Budgeting isn’t binary. It’s never the case that there’s some number that will guarantee success or failure.
- As a program leader, you are often going to be on both sides of the budgeting process: creating your own budget and evaluating other people’s proposed budgets.
- You should adjust your budget estimates up or down depending on who you need to attract to be successful. One aspect of “who do you need?” is how in-demand the skillset is: if you’re doing a program in an obscure area where experts are in low demand, you don’t need as much money as doing an AI project in 2024. Another aspect is how sensitive a project’s success is to the skill of the people executing it: do you need a team in the 99th percentile or could a 95th percentile team do it? Adjust budget estimates accordingly.
- In the world of budgeting programs, you might hear the following acronyms:
- ROM — rough order of magnitude
- WAG — Wild-ass guess
Red Flags
If you see these in a budget, you should be wary. Similarly, you shouldn’t do these when budgeting!
- Too many significant figures. Based on the org you want to round to the nearest $1/2/5M.
- When a PI is going to be spending less than 20% of their time on a program.
- If the common assumption is that a project will take N people X months and someone wants to use 2N people and take X/2 months, they probably will not succeed.
- Linear spending — if someone budgets the same amount for every unit of time.
An aside on top-down budgeting
The reality is that a lot of your budgeting considerations are going to be driven less by how much the program would cost in a vacuum and more by where the money is coming from. As a result, budgeting is inextricably tied to fundraising (whether from external funders or higher levels within an organization). Some considerations for top-down budgeting:
- Figure out both the ceiling and normal amount of money that an organization deploys into a program.
- When asking for money, don’t be shy! Chance of rejection doesn’t actually change with the amount you’re asking for as long as it's well-reasoned and under the organization’s ceiling.
- Related to the above, standard negotiating tactics apply: you want to anchor your counterpart on the highest amount that won’t cause them to leave the negotiation.
- Understand the dynamics of money within the organization:
- Does the organization have a hard budget that can be exhausted? If so, how much is left?
- Does the organization have a budget it wants to spend down by the end of the year? If so, how much do they want to unload?
- What are the rules around things that can be funded within the budget you’re asking for? Can you pay for travel? Meetings? Small seed projects? Or are those things funded separately?
Institutional moves for interfacing with other organizations
Note that none of this is legal advice!
There are many ways to get external work done in a coordinated research program: everything from soliciting proposals and giving grants to the best proposals to hosting a competition to hiring someone to do a specific piece of work to convincing two other organizations to sign a joint development agreement with each other. You could think of each of these arrangements to get one or more people or organizations to do a thing as an "institutional move." Because you aren't doing any of the work with your own hands, institutional moves are your tools for getting things done.
Grants vs. Contracts
Many institutional moves bottom out in giving an organization or individual money. Money can go out the door in the form of either grants or contracts, so it's good to be familiar with what each entails. Broadly, the difference between a grant and a contract:
-
Grants
-
Scope of work is defined by the applicant
-
No legally binding requirement to achieve results
-
Assumes "best effort" on the part of the applicant
-
Enables more flexibility in outcomes beyond what was initially agreed upon
-
IP generally is owned by the public or creator
-
-
Contract
-
Scope of work is defined by the buyer
-
Legally binding agreement to provide specific goods and services in exchange for compensation
-
IP is generally owned by the buyer
-
Here is a more detailed table of differences in the US government and here is an article about the differences.
Some potential institutional moves
Theoretically, there are an infinite number of potential institutional moves. Which moves you can actually execute on are limited by:
-
Imagination
-
Legality
-
Requirements of your particular organization and their contracting authority
And of course, what other people are willing to agree to.
Some more common moves:
-
Giving a grant to an academic organization.
-
Grants don't give you any power over the output or intermediate products
-
Once you've given a grant, it is incredibly hard to get it back
-
You need to negotiate both with the PI and the university
-
The work will primarily be done by graduate students
-
-
Working through a prime contractor who breaks up work into subcontracts
-
Signing a contract with an organization for a specific work product
-
Giving a grant to a startup.
-
Giving a grant to a nonprofit organization
-
Creating a competition with a prize pool
- Winnowing down competitors
-
Running a series of hackathons
-
Stipulating that two organizations need to integrate components
-
Having one organization generate some kind of result and have another organization test those results
-
Creating a consortium of organizations through some combination of convenings and putting in initial money.
-
Creating a public dataset
-
Creating a set of standards or interfaces
Note that these moves are not mutually exclusive: you could (and often need to!) sign a contract with a contractor to run a competition in addition
Some less conventional moves that one could imagine:
-
Giving a postdoc or independent researcher a grant to do work at an academic lab or independent lab space.
-
Helping change regulations, potentially by providing experimental evidence
-
Matching funding from other private or government organizations
-
Creating clear tranches with milestones at which other organizations will put in funding
-
Setting up an endowment for an open source project
-
Doubling down on a project that is going surprisingly well
Knowing When to Quit and Setting Up Kill Criteria
One of the reasons that programs are able to take large risks is the willingness to kill off projects or entire lines of investigation. Quitting enables you to redirect time and resources towards more productive uses. Knowing when to quit is an art and actually doing it is incredibly hard, especially when it means firing employees or effectively firing external performers.
Quitting can happen at several points:
-
Abandoning an idea while designing a program.
-
Choosing not to continue a line of work after an initial experiment.
-
Ending a project before getting to a specific endpoint.
There are several reasons why you might quit:
-
You realize (through analysis or experiment) that an idea violates the laws of physics.
-
You determine that an approach won't scale or is otherwise a dead end.
-
A problem is primarily a non-technological issue.
-
Other work has made a project or approach obsolete.
-
An external collaborator just isn't good or shows themselves unable to execute.
-
Someone higher up decides that they don't want to continue funding your program for several reasons -- anything from budget cuts to optics to politics.
Good kill criteria:
-
Are objective. The most straightforward way to achieve objectivity is measurability, but requiring measurability can rule out many good projects.
-
Are agreed upon by all parties involved. This often requires negotiation.
-
Have ways that employees or external collaborators can realize that they aren't on target and step up their game. This might look like intermediate checkpoints or a continuously variable metric.
Concrete tactics around quitting:
-
Create precise, falsifiable hypotheses.
-
Timebox everything --- whether it's your own exploration of ideas or performer projects.
-
Be very clear with everybody involved up front about what rate of progress you expect.
-
One way to make sure that you're on the same page with researchers (both internal and external) about expectations is to have them specify their own milestones (with some nudging towards ambition).
-
Create tight feedback loops with everybody involved in the program and especially researchers, both internal and external to the org.
-
Once you know that you should quit, act as fast as possible. Anything else is a waste of time and money.
Concrete Examples
-
Cutting sub-projects or external collaborators due to cost overruns. During the Miniature GPS Receiver Program, Tony Tether cut Magnavox as a performer because they had repeated cost overruns. Pruning performers based on cost overruns is not uncommon.
-
Cutting sub-projects or external collaborators that fail to hit milestones. During the F6 Program (Future, Fast, Flexible, Fractionated, Free-Flying Spacecraft United by Information Exchange) DARPA aimed to develop a new approach to satellite architecture. The program's PM had to make tough decisions around cutting performers who were not meeting the milestones in developing modular and flexible spacecraft systems.
-
Cutting teams from a staged competition based on milestones. During the DARPA Robotics Challenge teams had to succeed at one or two different sub-challenges (a competition with a simulated robot and a scoped version of the final challenge) to make it to the final competition. Those that failed at the challenges were cut and those that succeeded were given additional funding. (See image)
Examples of programs that ended early. (Note that it's also a reason to quit if you are convinced that a program would run into one of these situations during the design phase):
-
Quitting a program because the approach is deeply mismatched to the goals. The Fast Adaptable Next-Generation Ground Vehicle program was focused on building open-source vehicle design and manufacturing, but was ultimately ended early because there were enough classified military designs and materials that an open source approach just didn't work for military vehicles.
-
Quitting because it didn't seem possible to hit key metrics. The Exoskeletons for Human Performance Augmentation Program aimed to develop wearable exoskeletons to enhance soldier performance. It faced significant technical challenges, especially in power supply and weight reduction. The technological hurdles and the high cost of development led to its early termination.
-
The Transformer (TX) Program sought to develop a flying car-like vehicle for military use. It encountered numerous challenges in design and feasibility, particularly in achieving the desired performance within the constraints of size, weight, and power. The program was terminated early due to these technical and practical limitations.
-
Quitting because of politics. The Total Information Awareness (TIA) Program was developing technologies for large-scale data mining and analysis to prevent terrorist attacks. It was terminated due to public concerns over privacy and civil liberties. The controversy over surveillance and data collection led to a loss of support in Congress, resulting in its early closure.
-
Quitting because the world changes. The SyNAPSE (Systems of Neuromorphic Adaptive Plastic Scalable Electronics) program, started in the late 2000s, aimed to create electronic neuromorphic machine technology that would function similarly to a human brain. The goal was to develop a new generation of computers and electronics that could interpret, analyze, and learn from data in a sophisticated, efficient manner, much like the human brain. However, as the program progressed, the rapid advancement of machine learning and artificial intelligence in the broader tech industry, particularly the development and improvement of deep learning algorithms, overshadowed the goals of SyNAPSE. These advancements in AI and machine learning offered more immediate and practical solutions to problems SyNAPSE aimed to address.
Deep learning, leveraging large neural networks and massive amounts of data, began to demonstrate remarkable capabilities in image and speech recognition, natural language processing, and other areas that were once thought to require the kind of neuromorphic computing DARPAs SyNAPSE program was exploring. As a result, the focus in the tech industry shifted towards these more immediately applicable AI technologies, which were rapidly evolving and being integrated into various applications. This shift made some of the objectives of the SyNAPSE program seem less relevant, leading to its early wind-down.
Related resources:
Metrics
Metrics for coordinated research programs (and research in general) are incredibly tricky because good metrics are far more powerful than no metrics, while bad metrics can be actively harmful. (In a nutshell, good metrics > no metrics > bad metrics)
A note on terminology: This section will talk about both “metrics” and “quantifiable goals” because it’s hard to talk about one without discussing the other. The line between “metrics” and “quantifiable goals” is fuzzy but metrics have a sense of being measured continuously and used as indicators while goals have a sense of being measured only at specific times and being more binary in their outcomes.
What is the purpose of metrics?
Metrics can serve several purposes:
- Agnostically adjudicating between different approaches to a problem. A good metric can enable you to compare drastically different approaches and ultimately decide which is more promising.
- Introducing a new paradigm. Commonly used metrics often encode paradigms in a field or industry. Introducing a new metric can push people towards new paradigms. For example, a common metric in semiconductors is “price per transistor.” The industry touts how low that price is compared to other widgets. However, if you looked at the “price per mole of transistors” the price looks incredibly high compared to other things we use in bulk, like drugs.
- An internal barometer or dashboard of progress over time. Metrics are one way for program leaders and people on project leads to have a sense of how close they are to hitting their goals.
- Focusing and driving work. Metrics are a great way to make sure teams and collaborators are on the same page about what is important. Those same focusing metrics can also be a catalyst for conversations about what’s going on if they’re not being hit.
- An easy way for someone external to a project or program to judge progress and success. From the outside, it’s much easier to compare two numbers (target number and achieved number) than any other way of evaluating an effort you’re not inside of.
Ideally, all of these purposes will align behind a single, straightforward to measure metric for the program, perhaps with some project-by-project intermediate metrics. Unfortunately, that is almost never the case.
Different people and organizations will have different approaches to metrics, from using them as law to eschewing them entirely.
How to think about creating metrics
- Determine whether there is a metric that is an actual proxy for getting closer to the solution
- Example: if you’re trying to make better rockets, the metric of newtons of thrust per kilogram of mass in a thruster relates directly to how much mass the rocket can get into orbit, which is its primary purpose.
- Think seriously about whether a program or project lends itself to metrics. In large part, suitability for metrics has to do with the amount of uncertainty about what the final outcome looks like. The question of metric suitability is fraught: applying metrics to a situation where they’re inappropriate can lead to wasted work, but it’s also tempting to use that as an excuse just to avoid the restriction of freedom that metrics create.
- For any goal, ask whether hitting it will convince the people you want to care that something is possible or exciting. If not, it’s a bad goal.
- Example: if a program was trying to make a high tensile strength material for a space elevator but the process couldn’t make the material longer than a few centimeters, it won’t make people think a space elevator is any more possible than before. (This is the current situation with carbon nanotubes.)
- Ask “what intermediate metrics would let me know that we have gotten past a key risk?” These are the “~training the monkey~” parts of the program.
- Example: if you’ve identified that corrosion on an electronics component is a key risk, using contact resistance as an intermediate metric is a good idea.
- Determine (at least for yourself) whether a goal is a “must hit” or a stretch goal meant to drive effort.
- Example: if you’re trying to make a wall-penetrating radar and would be happy if it could penetrate one meter of concrete, you might want to set a stretch goal of being able to penetrate two meters of concrete. However, if you’re making a backpack mounted device, weighing less than a person can carry is a must-hit goal.
- Cost or system scalability are legitimate metrics! Many times, bringing the cost of a process down is a research-heavy activity and is what will actually get someone to care.
- Use as few metrics as you can but no less. Having too many metrics can lead to not focusing on what’s actually important, but too few metrics can end up ignoring those important things!
- Understand what metrics you need to hit for a technology to transition.
- Example: a DARPA program to create artificial blood hit all its technical metrics, but the artificial blood was so much more expensive than blood from a blood bank that the technology was never used.
Metrics for a program’s overall success
It’s especially tricky to create metrics for the overall success of a coordinated research program for two reasons:
- Their impact almost always happens on the timescales of years or longer.
- Often, their impact happens in unexpected ways.
That being said, it’s useful to be able to point to some concrete indicators.
Obviously, one metric for overall success is “did you hit your goals and intermediate metrics?” This is one of the few metrics that can be assessed immediately at the end of the program. Of course, judging a program solely on hitting its goals assumes both that you selected the right goals to have an impact and that unexpected factors won’t affect the amount that even the right goals can have on impact.
Some other potential metrics that could be used at time intervals after the program ends:
- Follow-on money that goes towards work that follows the program: this could include startups, nonprofits, and research programs in both governments and large companies.
- Use of a term coined during the programs.
- Contributions to or uses of tools or resources the program creates.
- Papers and patents that come out of the program and citations of those publications.
Two caveats around program success metrics:
- The same metrics that indicate a program’s ultimate success or failure should not be used as intermediate milestones. Projects will rarely show continuous improvement.
- Beware that all of these metrics can warp the program if you emphasize them too much. (See what has happened to academia with the rise of citation metrics!)
Metrics failure modes
- Creating a single compound metric out of a number of other metrics: when there are a number of different measurements you care about, it’s tempting to assign each one a weight and create a single compound metric. This is a bad idea. In addition to it being almost impossible to pick the right weights, compound metrics often obscure critical flaws.
- ~Goodheart’s law~: people are incredibly good at optimizing for a metric when they have enough incentive to do so. If you emphasize a proxy metric for something less measurable, it’s easy to wind up in a situation where you’re maximizing that metric without addressing the thing it's a proxy for.
- Not updating a metric or goal when new information comes up: not infrequently you will discover something in the course of a program that makes a metric or measurable goal irrelevant to the goal of the program. It’s a hard conversation with stakeholders and collaborators to tell them that you were wrong about the original metrics and need to change, but sticking to the old metric will lead to failure.
- Focusing on a metric at the exclusion of all other sorts of information about a program’s success: many important things cannot be quantified.
- Creating a metric in a situation where there’s too much uncertainty to know what the right metric is.
- Expecting a metric to show continuous improvement: especially in research, progress is often nonlinear. A metric can show no movement towards a goal for a long time and then suddenly explode when someone has a breakthrough.
- Trying to quantify something that isn’t actually a quantity: it’s tempting to create quantifiable proxies for non-quantitative things like “usability.” These proxies almost always fail to drive work in a useful way.
Some examples of good metrics
- Strength to weight ratio of a material (and really any physical properties)
- Yield of a process
- Number of components in a system
Some examples of bad metrics
- Number of collaborations
- Lines of code
- Media mentions
- Number of publications (without any other consideration)
How to Write a Good Solicitation
Specifics of how you write a solicitation will depend heavily on how your organization does things and the design of your program. Here are some generally applicable tips:
-
Spend more time on what you are not looking for than what you are looking for
-
Leave yourself open to approaches you haven't imagined. Two ways to do this are
-
Focus on what you are not looking for (see above)
-
Be very clear that your goals and metrics are directly aimed at the problem you're trying to solve or the capability you're trying to build. It's easy for metrics to assume a specific paradigm --- as a made-up example, if a program was focused on incredibly efficient vehicles, asking for a motor that can get 100 miles/gallon would rule out electric or fuel-cell vehicles which might actually accomplish the goal better.
-
-
Put out a call for short (~2-page) letters of intent (LOIs) before a call for full proposals. The call for an LOI should look roughly like the full solicitation except asking for shorter documents and fewer details (eg. No need for a detailed budget or timelines). Doing an LOI call first has several advantages:
-
It saves everybody time. Ideally the majority of the full proposals will be good because they got a go-ahead from you. That way, you don't have to waste a lot of time reading long proposals and people who would be rejected don't have to spend time writing them.
-
It enables you to refine the full solicitation based on common misunderstandings or things you didn't see but would like to see more of in LOI submissions.
-
Similar to #2, it enables you to see which groups of people/disciplines/labs didn't submit LOIs that you would have wanted and then go encourage them to submit something.
-
An effective LOI makes the full proposals more like a contract negotiation than a lottery, which is much kinder to already-stressed PIs.
-
-
Regardless of whether you have a LOI or not, you ideally have in mind several real people who you would want to respond to while writing the solicitation. More specific audiences let you write tighter solicitations and reduce confusion.
A rough outline of a good solicitation
-
Title. Create a concise and descriptive title --- the title should be concise yet descriptive, capturing the essence of the program. Obviously there is tension between descriptive and concise!
-
Good Example: "Advancing Autonomous Systems in unstructured environments"
-
Bad Example: "Next-gen AI: AI systems anywhere anytime for anybody that can operate in situations where other AIs cannot" (This is both long and unspecific )
-
-
Goals. Before anything else, state the program's specific and ambitious goals in the most simple language possible. It's tempting to give background first or go very detailed but do not do this!
- Example: "Develop AI algorithms capable of real-time decision-making in dynamic environments."
-
Background. Give readers brief and relevant context for why the program exists in the first place. Keep it short and directly related to the objective.
- Example: "Current AI systems struggle in unstructured environments. This program seeks to address this gap. <Some more information about the specific failure points and contexts of use you are hoping to address>
-
Scope. Outline specific areas of interest or research topics. This guides potential contributors towards relevant proposals. Keep the scope defined and focused, while leaving yourself open to unanticipated approaches or ways to achieve the program's high-level goals.
- Example: "Focus areas include hardware-software co-development, transfer between simulation-based reinforcement learning and live sensor-in-the loop learning, and human-AI collaboration."
-
Requirements. List specific criteria that proposals must meet. Clarity here prevents ambiguity and ensures quality submissions. Ideally, these are as clear and measurable as possible.
- Example: "Proposals must demonstrate a pathway to achieving real-world application within five years." <List of indicators of this>
-
Timeline. Provide a timeline with distinct phases and milestones. This shows the program's trajectory and expectations. Ideally, this timeline is as realistic and structured as possible.
- Condensed Example: "Phase 1 (12 months): Prototype development. Phase 2 (24 months): Field testing."
-
Budget. Clearly state the budget. Providing guidelines on funding per project helps in planning and proposal alignment. The budget should be as transparent and justified as possible.
- Example: "Total budget is $10M, with individual project funding up to $2M based on scope and impact."
-
Proposal Format. Specify the format and content for proposals. This ensures uniformity and ease of evaluation. This format will depend heavily on your organization, but wherever you have leeway, think about how you can make the proposal as short as possible while still giving you enough information to make a decision.
- Example: "Proposals should follow the provided format: Executive Summary, Technical Approach, Team Qualifications, Budget. No more than one page per section."
-
Evaluation Criteria. Define how proposals will be judged. This guides applicants in structuring their proposals effectively. These criteria should be as objective and comprehensive as possible.
- Example: "Proposals will be evaluated based on how directly they address the capabilities this program is trying to build, counterfactual impact if the project is successful, clear timelines, and whether the project would be able to get funding from other sources.
-
Submission Guidelines. Make sure that people know how and when to submit their proposals. It's organization dependent but ideally the submission is as straightforward and accessible as possible.
- Example: "Submit proposals via the online portal by . Late submissions will not be accepted."
-
Contact Information. Make sure potential applicants have a point of contact for questions or clarifications. Answer questions promptly!
- Example: "For queries, contact the program coordinator at ."
Remember, the key is to be clear, concise, and focused. Your solicitation should inspire and guide potential contributors, providing them with all the necessary information to submit high-quality, relevant proposals.
There are many examples of ARPA solicitations available at https://grants.gov/search-grants (filter for your favorite ARPA Office)
Coordinated Research Leader Archetypes
Different coordinated research goals require very different kinds of leadership. Different people are also inclined towards different leadership styles. (It's worth noting that successful programs require a match between the style of leadership a program needs and the style of leadership the program's leader is inclined towards, but there is flexibility on both sides.)
Calling out specific archetypes is incomplete by its nature. Any individual program leader will rarely fall exactly in any given archetype --- you could think of these archetypes as basis vectors for approaches to program leadership.
None of these archetypes are "better" or "worse":
Grand visioneer
The grand visioneer leads through an inspired vision for radical change that's able to inspire others. They are able to get their vision into other people's heads to the extent that they adopt it as their own. A grand visioneer gathers the best talent who have bought in, makes sure they know each other, and gives them the resources to pursue their ideas with minimal interference.
Good for
- Creating new fields and exploring a technology landscape.
Bad for
-
Situations that need specific implementation pathways
-
Short impact timelines
-
Situations that need tight coordination
-
Micromanagers
Tactics
-
Creating vision documents
-
Consistent workshops
-
Enabling people who have not yet established themselves
-
Helping start labs
Examples
Managing change agent
A managing change agent identifies a concrete step change that can be completed within the lifetime of a program or two. The managing change agent gathers the best talent in a "structure" and monitors them to make sure that they're moving towards a specific goal.
Good for
- Finalizing groundbreaking prototypes/products.
Bad for
-
Broad, fuzzy ideas.
-
Creating new fields.
Tactics
-
Creating an architecture and specifications and giving specific tasks to different groups
-
Competitions
Examples
-
Bob Kahn when creating TCP/IP
-
Norm Whitaker who ran the second DARPA grand challenge
System change agent
A system change agent creates and stewards an unintuitive but carefully constructed vision based on deep knowledge of a critical system. They have a deep relationship to market and industry trends and are able to generate consensus among complex and/or conflicted stakeholders by communicating openly and giving clear guidance.
Good for
-
Creating unintuitive or unsexy advances that will have large ripple effects
-
Making advances in complex or opaque industries
-
Changes that need an industry to get on board
-
People who have deep industry background
Bad for
-
People without existing networks in an field, industry, or discipline
-
Fragmented or nonexistent ecosystems
Tactics
-
Workshops and meetings to build consensus around
-
Joint development with existing players
Examples
Finisher
Finishers are managers of sort-of-known paths (not creators of new paths). They manage to goals, time, cost, impact. They get sh*t done. This archetype is perhaps the least "sexy" but potentially the most impactful.
Good for
-
Situations where all the pieces are there but nobody is putting them together
-
Situations where "everybody knows" what should be done but it is hard/expensive/not incentivized/unpopular
Bad for
- People who want to work on their own new ideas
Tactics
-
Parallelized efforts and cutting performers who aren't performing.
-
Strong project management: Gantt charts, etc.
Examples
Captain Kirk
Captain Kirks are people who can innovate in a very fast moving or chaotic area with critical but often highly uncertain possibilities and risks. The AI/LLM world of 2023 is a good example of such an area. They have the ability to read the world, credibly predict/articulate trends in a fast changing domain with lots of uncertainty, and then figure out what is not happening that could be additive to the whole field.
Good for
- Areas where there is already a lot of action
Bad for
- Areas that have been stagnant
Tactics
-
Fast, small, derisking projects
-
First principles thinking
Examples
-
Geoffery Ling --- HAND (neurotech + biotech work)
-
Justin Sanchez (former director BTO + 3 neurotech programs)
PI of PIs
The PI of PIs acts something like a principal investigator (PI) but instead of grad students and postdocs working under them, there are other PIs. Here "acting like a PI means co-designing projects with collaborators/grantees/performers towards a larger vision, holding "lab meetings" to coordinate, all with varying level of hands-off-ness. A PI of PIs roughly stays within and work across their previous field (viewed broadly).
Good for
-
Established researchers in an area.
-
Existing fields.
Bad for
-
People with less prestigious track records.
-
People coming into an area from the outside.
Tactics
- Regular coordination meetings.
Generalist
The generalist sees a solution or capability that falls outside of any established field. They pull together people from very different worlds to work together and help translate between them.
Good for
-
People who have training in a discipline that lends itself to first-principles thinking like physics.
-
Situations where there are experts across several disciplines who should be collaborating who are not.
Bad for
- People who are not deeply technical.
Tactics
-
Creating new networks very quickly.
The Enabler {#enabler}
The enabler acts as a salesperson and advocate for other researchers ambitious ideas rather than building a program around their own ideas.
Good for
-
Great salespeople
-
People who want to have an impact but don't have a strong thesis
-
Areas with lots of small bottlenecks instead of single large ones
Bad for
- People who like to be steering the ship
Tactics
- Talking to and identifying the top people in a field
Examples
- Ken Perko
Glossary
This playbook was originally written specifically for ARPA-style programs. It has been expanded to apply to coordinated research programs more generally. As a result, it still uses some ARPA-related jargon. It also uses some general terms that correspond to ARPA jargon. This glossary is meant both to introduce people unfamiliar with the ARPA world to the jargon in the playbook and to orient those familiar with ARPA terms to their counterparts in the playbook.
-
ARPA-style programs are programs in the style of DARPA and organizations modeled after it (like ARPA-H, ARPA-E, IARPA, etc.) The defining characteristics of an ARPA-style program is that it's led by an empowered program manager, does work entirely through external researchers, has ambitious goals, lasts ~5 years and deploys ~$50M. See here for much more detail.
-
Focused research organizations are time-bound, non-academic research organizations focused on building a specific tool, dataset, or piece of knowledge. See here for more.
-
Internal vs. External work refers to whether the actual work (pipetting, coding, drilling, etc) is done within the organization coordinating the research or not. In an ARPA context, all work is external work.
-
External collaborators are people or organizations who are doing research work as part of the program but are external to whatever organization is running the program. In an ARPA context, they would be called "performers." External collaborators could be companies (of many flavors), academic labs, or individuals. There's a wide range of ways that collaborations can be structured, from informal agreements to grants to contracts with clear deliverables.
-
Projects are activities with a specific deliverable. A program is made up of one or more projects.
Acknowledgements
There are many many people whose wisdom and work contributed to this playbook. As a synthesis, inevitably I will have absorbed some ideas without even realizing who originated them. Apologies in advance for that! As a hopefully living synthesis, may this list be ever expanding.
Thanks to:
ARIA, for supporting the foundational work underpinning this playbook.
Jessica Alföldi, for meticulous editing and tireless enthusiasm for the whole project.
The massive number of people with far more first-hand experience than me who talked through these things:
Joshua Elliott, Ilan Gur, Adam Russell, Steve Buschesbaum, Arati Prabhakar, Adam Marblestone, Bridgett Baumgartner, Geoff Ling, Tom Kalil, Patrick McGrath, Amy Kruse, Brad Ringeisen, Dan Wattendorf, David Gunning, Eric Van Gieson, Hemai Parthasarathy, John Blitch, Mark Micire, Mike Fiddy, Pae Wu, Phil Root, Wade Shen, Lisa Porter.
License
The Research Leader's Playbook by Speculative Technologies is licensed under a Creative Commons Attribution 4.0 International License.
To view a copy of this license, visit http://creativecommons.org/licenses/by/4.0/
The Right People with the Right Ideas.
Subscribe to our newsletter to stay in the loop.
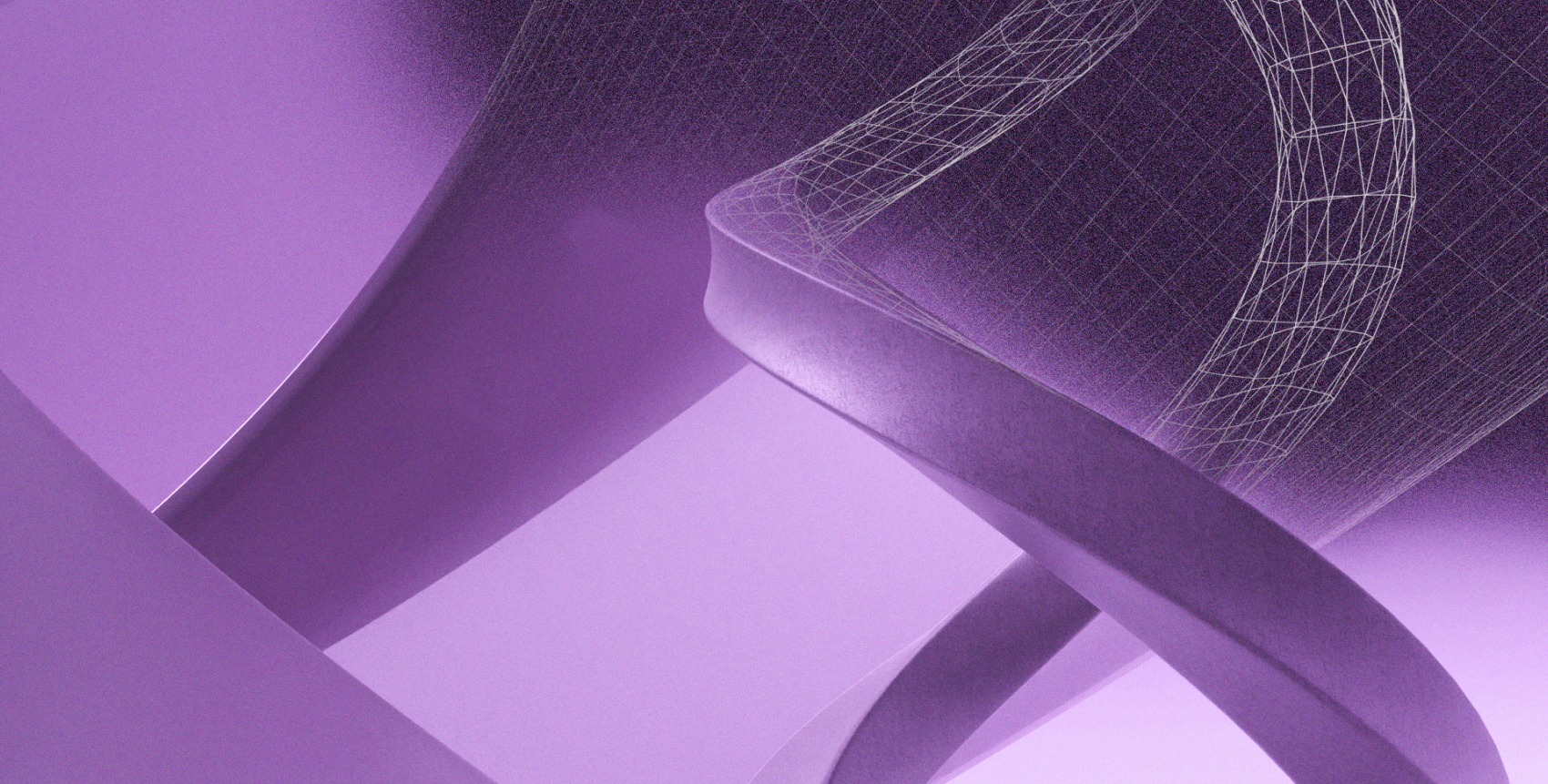